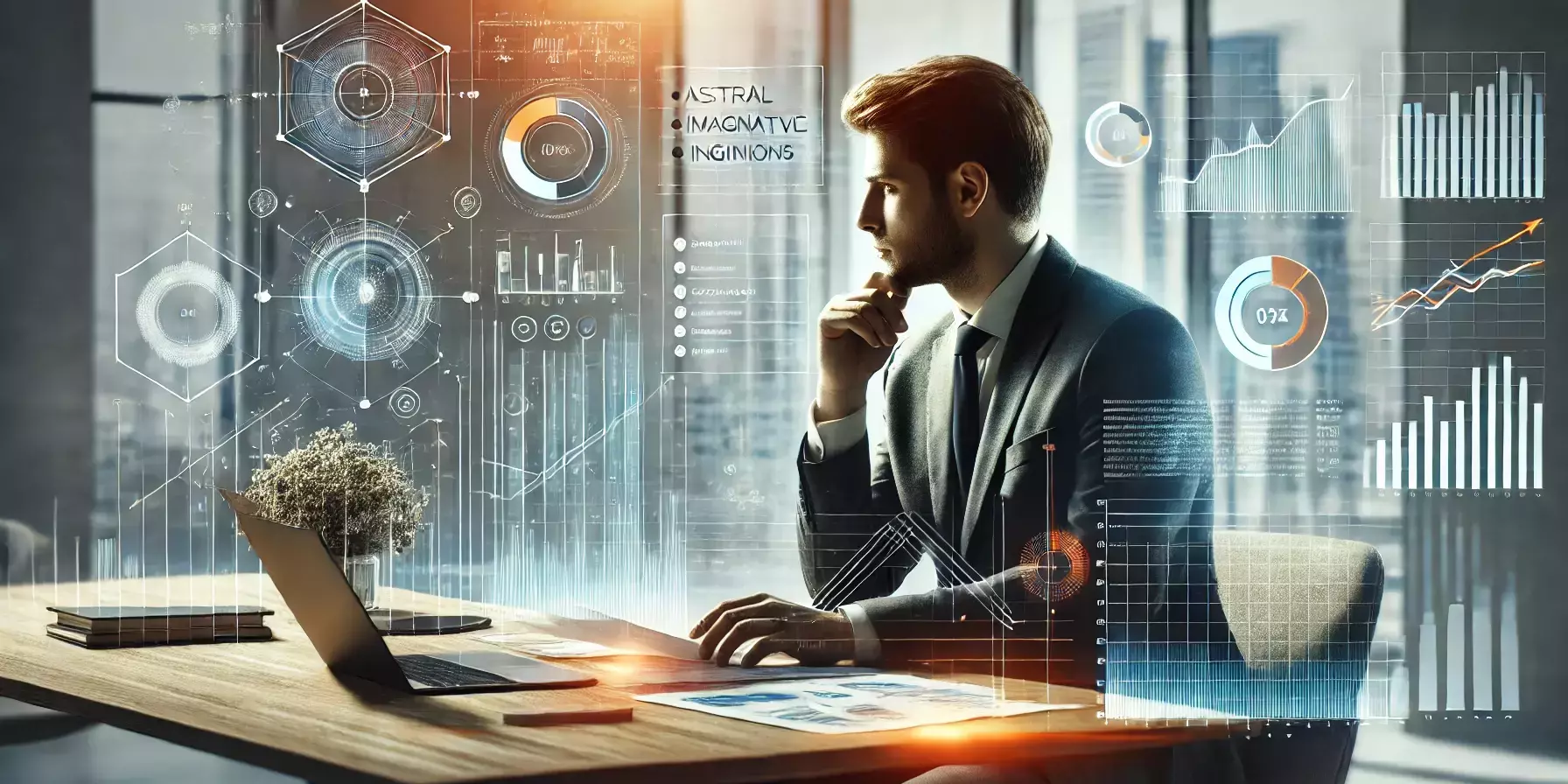
In an era where businesses are inundated with data, making informed decisions quickly and accurately has never been more crucial. Traditional decision-making processes often struggle to keep pace with the complexity and speed of modern markets. Enter AI-driven decision support systems (DSS)—a transformative technology that empowers businesses to make smarter, faster, and more efficient choices.
For early adopters navigating Geoffrey Moore’s Crossing the Chasm model, embracing AI-driven DSS offers an opportunity to lead their industries, enhance productivity, and unlock unparalleled efficiencies. This article delves into how AI-driven DSS works, its benefits, and actionable strategies for implementation.
What Are AI-Driven Decision Support Systems?
AI-driven DSS combines artificial intelligence (AI) with traditional decision support tools to process vast amounts of data, generate insights, and recommend actions. Unlike static systems, these tools use machine learning (ML) and predictive analytics to continuously learn and improve, enabling businesses to:
- Analyze complex datasets quickly and accurately.
- Identify patterns and trends that inform strategic choices.
- Simulate scenarios to evaluate potential outcomes.
By automating and augmenting decision-making, AI-driven DSS transforms how businesses approach challenges and opportunities.
Key Benefits of AI-Driven Decision Support Systems
1. Enhanced Decision Accuracy
AI-driven DSS eliminates guesswork by providing data-backed recommendations. It identifies correlations, anomalies, and trends that human analysis might miss.
- Example: A retail chain uses AI to predict customer buying patterns, optimizing inventory levels and reducing stockouts.
- Impact:
- Fewer errors in strategic planning.
- Improved confidence in decision-making.
- Higher ROI from data-driven actions.
2. Increased Productivity
Automating data analysis and report generation frees up time for employees to focus on high-value tasks. AI-driven DSS reduces the manual workload associated with gathering and interpreting data.
- Example: An HR department uses AI to analyze employee engagement survey results, identifying areas for improvement in minutes rather than weeks.
- Impact:
- Faster decision-making processes.
- Reduced workload for teams.
- Streamlined workflows across departments.
3. Proactive Problem-Solving
AI-driven systems leverage predictive analytics to anticipate issues before they arise, enabling businesses to take proactive measures.
- Example: A logistics company uses AI to forecast delivery delays based on weather patterns and traffic data, adjusting schedules accordingly.
- Impact:
- Minimized disruptions.
- Increased customer satisfaction.
- Reduced operational risks.
4. Scalability Across Business Functions
AI-driven DSS is versatile, scaling to address challenges across various departments, from marketing to operations.
- Example: A manufacturing firm integrates AI into production planning, marketing strategies, and customer service.
- Impact:
- Consistent improvement across functions.
- Greater adaptability to market changes.
- Unified decision-making across the organization.
How AI-Driven DSS Enhances Productivity and Efficiency
1. Real-Time Insights for Faster Decisions
AI-driven DSS processes data in real time, providing up-to-the-minute insights. This capability is invaluable in fast-paced industries where delays can mean missed opportunities.
- Example: A financial services company uses AI to monitor market conditions and provide instant recommendations on asset allocation.
- Tools to Explore: Tableau with AI integrations, Power BI, and Qlik Sense AI.
2. Scenario Simulation and Risk Analysis
AI enables businesses to simulate scenarios and evaluate potential risks, empowering leaders to make informed choices.
- Example: An energy company uses AI to simulate the impact of different renewable energy investments on long-term profitability.
- Tools to Explore: IBM Decision Optimization, Microsoft Azure Machine Learning, and custom DSS solutions.
3. Customized Recommendations
Custom AI models tailor insights and recommendations to a company’s unique needs, ensuring relevance and applicability.
- Example: A healthcare provider uses AI to recommend personalized treatment plans based on patient data and medical history.
- Tools to Explore: Custom-built AI models using TensorFlow or PyTorch.
Steps to Implement AI-Driven Decision Support Systems
1. Define Clear Objectives
Start by identifying the key decision-making challenges your business wants to address.
- Example: Reducing supply chain inefficiencies or improving customer segmentation.
2. Select the Right Technology
Choose AI-driven DSS tools that align with your goals, budget, and existing infrastructure.
- Example: Use cloud-based AI platforms like Google Cloud AI or AWS Machine Learning for scalability and flexibility.
3. Integrate Seamlessly
Ensure the DSS integrates with existing systems, such as CRMs or ERPs, to streamline data flow.
- Example: Integrate DSS with Salesforce for enhanced sales forecasting and pipeline management.
4. Train and Upskill Teams
Invest in training to ensure employees understand and can leverage the DSS effectively.
- Example: Conduct workshops on data interpretation and AI-driven decision-making tools.
5. Monitor and Refine
Continuously evaluate the DSS’s performance and refine algorithms to improve accuracy and relevance.
- Example: Regularly update machine learning models with new data to maintain effectiveness.
Real-World Success Stories
1. Retail Sector
A global retailer implemented AI-driven DSS to optimize pricing strategies across regions. By analyzing competitor pricing, local demand, and customer behavior, the system increased profit margins by 15%.
2. Healthcare Industry
A hospital used AI-driven DSS to allocate resources during peak demand periods, reducing patient wait times by 20% and improving staff efficiency.
3. Manufacturing
A factory leveraged AI for predictive maintenance, saving $1 million annually by preventing unexpected equipment failures.
The Future of AI-Driven DSS
AI-driven DSS is evolving rapidly, with emerging trends set to redefine how businesses make decisions:
- Augmented Intelligence: Combining human expertise with AI insights for more nuanced decisions.
- Explainable AI: Ensuring transparency in AI recommendations to build trust among stakeholders.
- Federated Learning: Enabling businesses to collaborate on AI model training without sharing sensitive data.
For early adopters, these advancements present exciting opportunities to stay ahead of the curve.
Conclusion: A Smarter Way to Decide
AI-driven decision support systems represent a new era of productivity and efficiency for businesses. By automating data analysis, simulating scenarios, and providing tailored recommendations, these systems empower early adopters to make smarter, faster, and more impactful decisions.
The path to success begins with identifying your business’s unique needs, selecting the right tools, and fostering a culture of data-driven innovation. For those ready to lead, AI-driven DSS is the key to unlocking new levels of performance and staying ahead in a competitive market.
Embrace the future of decision-making — today.