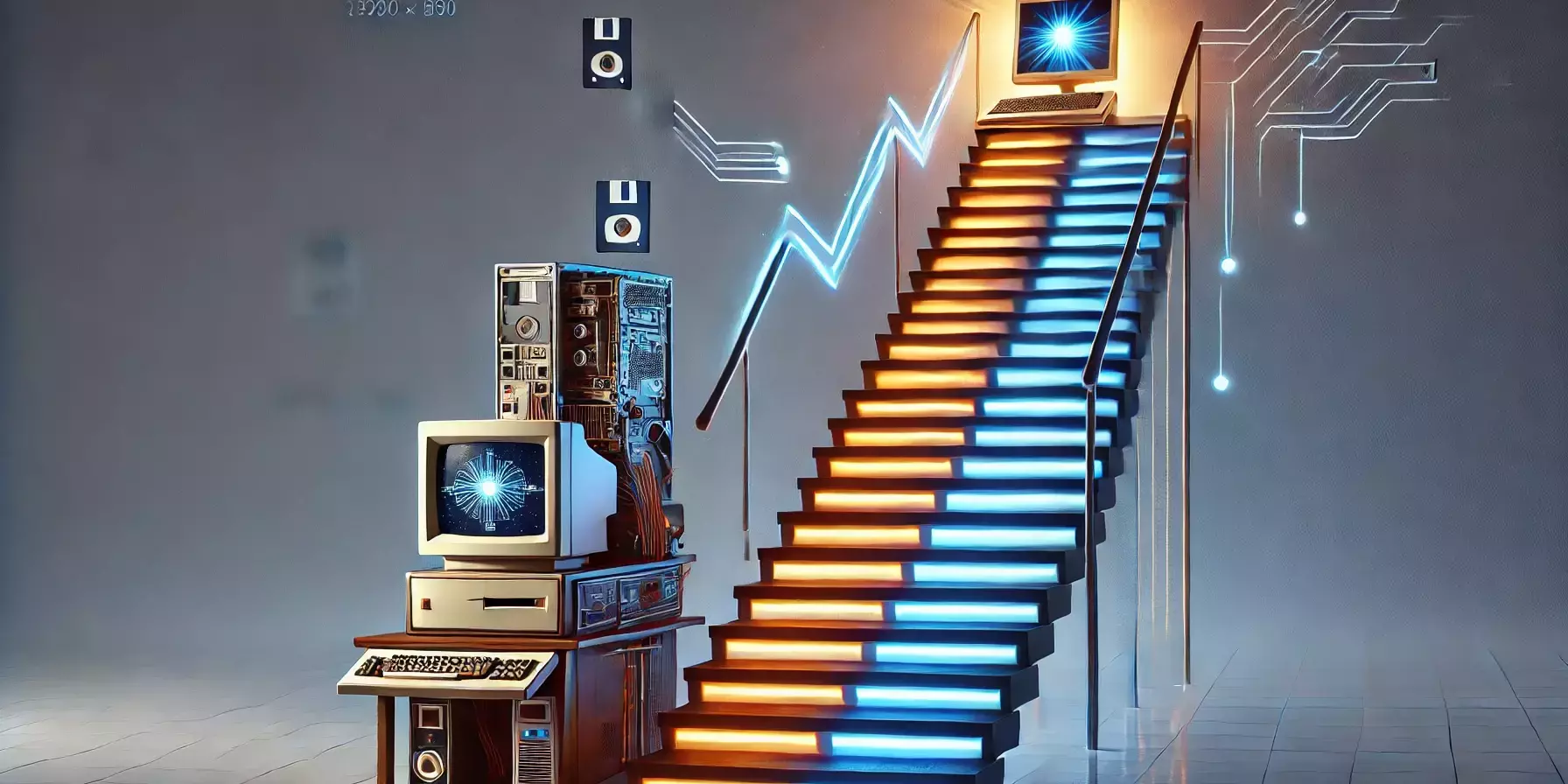
Artificial intelligence (AI) has become a transformative force, enabling businesses to innovate, optimize processes, and unlock new opportunities. However, implementing AI often involves integrating it into existing systems — a complex yet crucial step for ensuring its success.
For professionals looking to utilize generative AI, effective AI integration strategies are key to achieving value and driving adoption. This article provides a practical roadmap for integrating AI into existing systems, addressing challenges, and ensuring a seamless transition.
Why Integrating AI into Existing Systems Matters
AI is most impactful when it enhances and extends existing capabilities rather than replacing them entirely. Integrating AI into legacy systems allows businesses to:
- Leverage existing infrastructure: Reduce costs by building on current technologies.
- Minimize disruption: Ensure continuity while introducing advanced capabilities.
- Enhance functionality: Use AI to improve performance, automate tasks, and derive insights from data.
However, without a clear strategy, integration efforts can lead to inefficiencies, system conflicts, or wasted investments.
Step 1: Define Integration Goals
Before diving into technical details, it’s essential to clarify why you’re integrating AI and what you hope to achieve. Defining clear goals ensures that your AI initiatives align with business objectives.
Questions to Ask:
- What specific problems will AI solve?
- Which systems or processes will AI enhance?
- How will success be measured (e.g., efficiency gains, cost savings, customer satisfaction)?
Action Steps:
- Prioritize Use Cases: Identify high-impact areas where AI can deliver measurable results, such as improving customer support or optimizing inventory management.
- Engage Stakeholders: Involve team members across departments to ensure alignment on goals and expectations.
Step 2: Assess System Readiness
Integrating AI requires a thorough assessment of your current systems, data, and infrastructure. Understanding your starting point helps identify potential gaps and ensures smoother integration.
Key Areas to Evaluate:
- Data Availability: Do you have sufficient, high-quality data to train AI models? Are there gaps that need to be addressed?
- System Compatibility: Can your existing systems support AI tools? Will upgrades or middleware be required?
- Scalability: Can your infrastructure handle the increased processing demands of AI?
Action Steps:
- Conduct a Data Audit: Identify where your data resides, assess its quality, and address any inconsistencies.
- Evaluate Infrastructure: Determine if your systems need upgrades or if you should use cloud-based solutions like AWS, Google Cloud AI, or Microsoft Azure AI.
- Plan for Scalability: Ensure your infrastructure can scale as AI adoption grows.
Step 3: Choose the Right AI Tools and Platforms
Not all AI tools are created equal. Selecting the right solutions ensures compatibility with your existing systems and alignment with your business goals.
Considerations for Choosing AI Tools:
- Integration Capabilities: Does the tool integrate seamlessly with your current systems (e.g., CRM, ERP, or supply chain software)?
- Customizability: Can the solution be tailored to meet your specific needs?
- Vendor Support: Does the vendor offer robust support and training for successful implementation?
Action Steps:
- Explore APIs and Plugins: Look for AI tools with APIs or pre-built connectors for easy integration.
- Consider Open-Source Options: Tools like TensorFlow and PyTorch offer flexibility and cost-effectiveness for custom solutions.
- Leverage Industry-Specific Platforms: Choose platforms designed for your industry, such as Salesforce Einstein for CRM or Blue Yonder for supply chain management.
Step 4: Plan a Phased Integration Approach
AI integration should be incremental, starting small and scaling over time. A phased approach allows you to test functionality, identify challenges, and refine strategies without overwhelming your team or systems.
Phases of AI Integration:
- Proof of Concept (PoC): Implement AI in a low-risk environment to validate its feasibility and value.
- Pilot Program: Expand usage to a specific department or process, gathering feedback and refining the solution.
- Full-Scale Deployment: Roll out AI across the organization, ensuring proper support and training.
Action Steps:
- Define Milestones: Break the integration process into manageable phases, with clear objectives for each stage.
- Gather Feedback: Regularly collect input from users to address issues and optimize the solution.
- Iterate: Use lessons from earlier phases to improve subsequent deployments.
Step 5: Address Common Integration Challenges
AI integration often encounters challenges, from resistance to change to technical roadblocks. Proactively addressing these issues ensures a smoother transition.
Challenges and Solutions:
- Resistance to Change: Employees may fear AI will replace their roles or add complexity to workflows.
- Solution: Emphasize how AI enhances their work, offering training to build confidence and familiarity.
- Data Silos: Fragmented data sources can hinder AI effectiveness.
- Solution: Invest in data integration tools and adopt a centralized data strategy.
- System Incompatibilities: Legacy systems may not support AI integration.
- Solution: Use middleware or consider upgrading critical systems.
Step 6: Monitor, Optimize, and Scale
Integration doesn’t end at deployment. Continuous monitoring and optimization ensure that AI systems deliver ongoing value and adapt to changing business needs.
Monitoring Best Practices:
- Track Key Metrics: Measure AI performance against predefined KPIs, such as efficiency gains, error reductions, or cost savings.
- Gather User Feedback: Regularly collect input from employees and customers to identify improvement areas.
- Update Models: Ensure AI models remain relevant by retraining them with new data and adjusting algorithms as needed.
Action Steps:
- Establish Feedback Loops: Set up regular review cycles to assess performance and make adjustments.
- Scale Gradually: Expand AI integration to new areas only after demonstrating success in initial deployments.
Best Practices for Seamless AI Integration
To ensure successful AI integration, consider these overarching best practices:
- Start with Clear Goals: Define specific, measurable objectives for AI adoption.
- Involve Stakeholders Early: Engage employees, IT teams, and leadership throughout the process.
- Focus on Training and Support: Equip teams with the skills and resources they need to work effectively with AI tools.
- Embrace Agility: Be prepared to adapt and refine your strategy as challenges arise.
- Celebrate Successes: Share wins from early integrations to build momentum and enthusiasm for broader adoption.
Conclusion: Building a Future with Seamless AI Integration
For companies, integrating AI into existing systems is both a challenge and an opportunity. By following a structured, phased approach and addressing potential hurdles proactively, businesses can unlock the transformative potential of AI while minimizing disruption.
A thoughtful integration strategy ensures that AI enhances current capabilities, aligns with business goals, and drives measurable value. For those ready to embrace the future, seamless AI integration is not just achievable — it’s the foundation for sustained innovation and success.