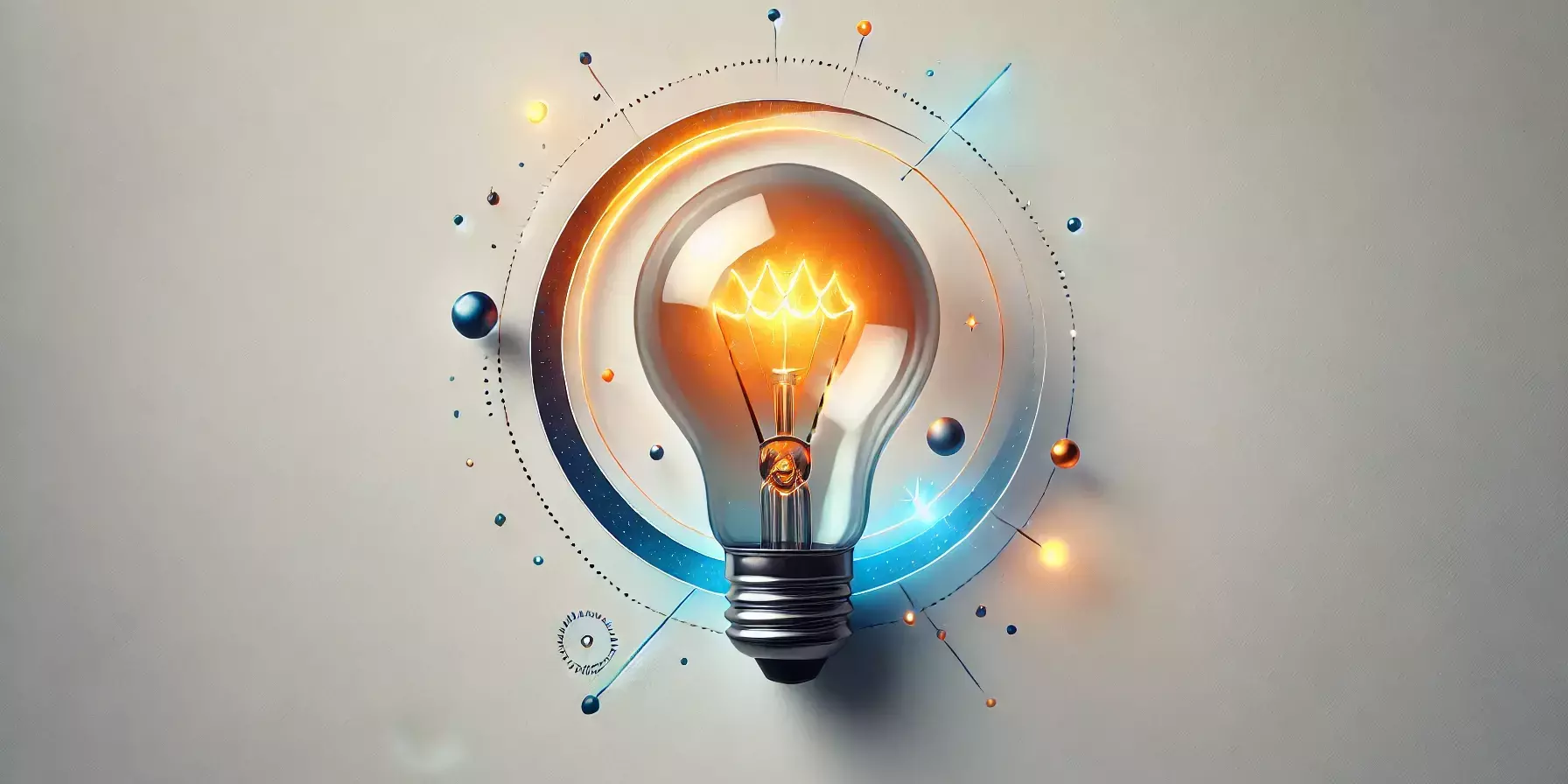
In a rapidly evolving digital world, artificial intelligence (AI) is no longer just theoretical — it’s a powerful tool driving business transformation. By automating processes, optimizing workflows, and generating actionable insights, AI is revolutionizing industries and delivering measurable results. For early adopters, understanding how businesses are successfully leveraging AI can provide a roadmap to innovation.
This article explores real-world case studies that showcase how AI-powered efficiency is reshaping businesses across industries, delivering tangible success stories, and inspiring new opportunities.
Why AI-Powered Efficiency Matters for Early Adopters
Efficiency is the cornerstone of business success. It drives cost savings, improves customer experiences, and enables organizations to remain competitive. For early adopters of AI, the potential to unlock new efficiencies often lies in:
- Automating repetitive tasks: Reducing manual workloads to free up time for strategic initiatives.
- Enhancing decision-making: Using AI insights to make data-driven, timely decisions.
- Optimizing resource allocation: Ensuring that people, time, and capital are used effectively.
By learning from businesses that have successfully integrated AI, early adopters can identify opportunities to improve their own operations.
Case Study 1: AI Streamlines Supply Chain Operations for a Global Retailer
The Challenge:
A global retailer faced inefficiencies in its supply chain, including delays in inventory restocking and unpredictable logistics costs. These issues led to lost sales opportunities and customer dissatisfaction.
The Solution:
The retailer implemented an AI-powered supply chain management system that analyzed historical data, real-time demand patterns, and external factors like weather and market trends. The system optimized inventory levels, predicted demand spikes, and recommended efficient shipping routes.
Results:
- 30% reduction in logistics costs: By optimizing shipping routes and minimizing fuel consumption.
- 50% improvement in inventory turnover: Ensuring products were available when and where customers needed them.
- Increased customer satisfaction: Faster restocking reduced the likelihood of out-of-stock items.
Case Study 2: AI Enhances Customer Support for a Financial Services Firm
The Challenge:
A leading financial services firm struggled to handle high volumes of customer inquiries, leading to long response times and inconsistent service quality.
The Solution:
The firm deployed an AI-powered chatbot integrated with natural language processing (NLP) capabilities. The chatbot answered common customer questions, assisted with account inquiries, and escalated complex issues to human agents.
Results:
- 60% reduction in response times: Customers received instant answers to routine queries.
- Improved agent productivity: By automating repetitive tasks, human agents could focus on resolving more complex issues.
- Enhanced customer experience: Faster resolutions and 24⁄7 support boosted customer satisfaction scores by 40%.
Case Study 3: AI Optimizes Manufacturing Processes for an Automotive Company
The Challenge:
An automotive manufacturer experienced frequent production delays due to unplanned equipment downtime and inefficient scheduling.
The Solution:
The company implemented an AI-powered predictive maintenance system that monitored equipment in real time. Sensors collected data on vibration, temperature, and other factors, which AI analyzed to predict failures before they occurred. Additionally, AI optimized production schedules to maximize efficiency.
Results:
- 50% reduction in unplanned downtime: Predictive maintenance minimized disruptions.
- 20% increase in production output: Optimized schedules improved throughput.
- $2M annual savings: Reduced maintenance costs and increased efficiency translated into significant cost savings.
Case Study 4: AI Drives Marketing Personalization for an E‑Commerce Platform
The Challenge:
An e‑commerce platform struggled to engage customers with generic marketing campaigns, resulting in low conversion rates and high customer churn.
The Solution:
The platform adopted an AI-powered recommendation engine that analyzed customer behavior, purchase history, and preferences. AI personalized marketing emails, product recommendations, and website experiences for each user.
Results:
- 25% increase in conversion rates: Tailored recommendations resonated more with customers.
- 15% reduction in churn: Personalized re-engagement campaigns retained more customers.
- Higher average order value (AOV): Personalized upselling and cross-selling strategies increased spending per customer.
Case Study 5: AI Enhances Fraud Detection for a Payment Processor
The Challenge:
A payment processor faced rising instances of fraudulent transactions, which threatened its reputation and customer trust.
The Solution:
The company deployed an AI-powered fraud detection system that analyzed transaction data in real time. The system used machine learning algorithms to identify anomalies and flag potentially fraudulent activities for further investigation.
Results:
- 90% accuracy in fraud detection: Reduced false positives and ensured legitimate transactions were not interrupted.
- $5M saved annually: Early fraud detection prevented financial losses.
- Improved compliance: Automated monitoring ensured adherence to regulatory standards.
Key Lessons from These Success Stories
These case studies highlight the versatility and impact of AI across industries. Here are the key takeaways for early adopters:
1. Start with High-Impact Use Cases
- Identify areas where inefficiencies are costing time, money, or customer trust.
- Example: Automating repetitive tasks like customer inquiries or inventory management delivers immediate ROI.
2. Leverage Data for Better Insights
- AI thrives on data. Ensure your organization collects, cleans, and leverages data effectively.
- Example: Predictive analytics in manufacturing relies on accurate sensor data for optimal results.
3. Focus on Scalability
- Pilot AI projects in specific departments or processes, then scale successful initiatives across the organization.
- Example: E‑commerce platforms can test personalized marketing on a segment before expanding to their entire user base.
4. Build Cross-Functional Teams
- Successful AI adoption requires collaboration between IT, operations, and business leaders.
- Example: AI fraud detection systems benefit from input from compliance teams and data scientists.
The Future of AI-Powered Efficiency
As AI technologies evolve, the potential for efficiency gains will continue to grow. Emerging trends include:
- Hyper-automation: Combining AI with robotic process automation (RPA) to automate end-to-end workflows.
- Edge Computing: Processing data locally for faster AI insights in industries like healthcare and IoT.
- AI-as-a-Service (AIaaS): Making AI accessible to small and medium-sized businesses through cloud platforms.
Conclusion: Transforming Businesses with AI-Powered Efficiency
For early adopters crossing the chasm, AI-powered efficiency offers an unparalleled opportunity to innovate, optimize, and lead in competitive markets. The success stories shared here demonstrate how AI transforms operations, reduces costs, and enhances customer satisfaction.
By leveraging AI strategically, businesses can unlock new levels of productivity and position themselves for sustained success in an AI-driven world. For those ready to embrace the possibilities, the future is bright — and incredibly efficient.