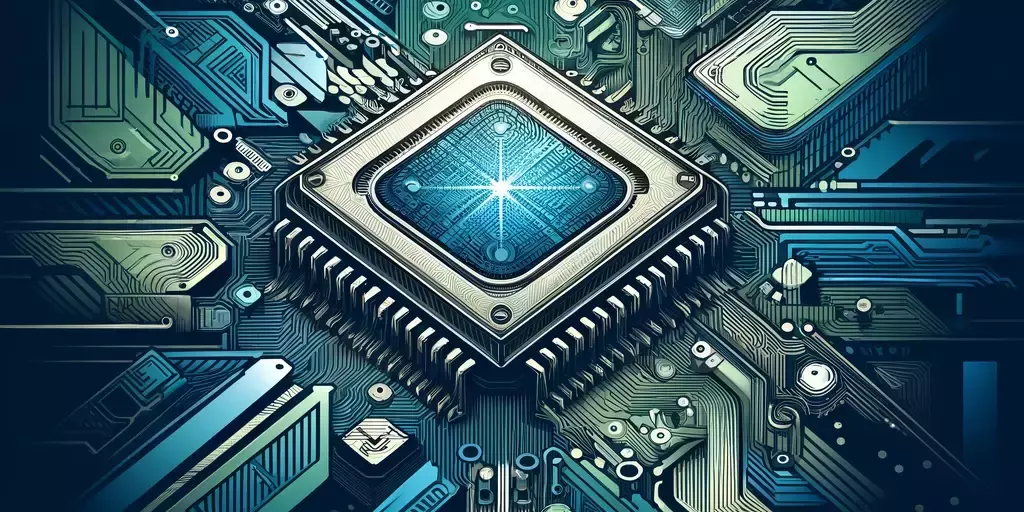
Artificial Intelligence (AI) is a broad term that encompasses various technologies aimed at mimicking human intelligence. Within this broad domain, Generative AI has emerged as a distinct subset with unique capabilities. In this blog, we will delve into the differences between traditional AI and Generative AI, highlighting their unique features, applications, and impacts.
Understanding Artificial Intelligence (AI)
AI is the overarching field that involves creating systems capable of performing tasks that usually require human intelligence. These tasks include learning, reasoning, problem-solving, understanding natural language, and perceiving sensory inputs.
Key Features of AI:
- Automation: Executes tasks without human intervention.
- Adaptability: Learns from data and improves over time.
- Versatility: Applies to diverse fields like healthcare, finance, and customer service.
Examples of AI Applications:
- Healthcare: AI algorithms analyze medical images to detect diseases.
- Finance: AI systems predict stock market trends and manage investment portfolios.
- Customer Service: AI chatbots handle customer inquiries, providing instant responses.
Understanding Generative AI
Generative AI is a subset of AI focused on generating new content from existing data. It uses complex algorithms to create text, images, music, and other forms of content that are not just a reproduction of the input data but original outputs that mimic the characteristics of the training data.
Key Features of Generative AI:
- Content Creation: Generates new and unique content.
- Pattern Recognition: Learns patterns from large datasets.
- Creativity: Produces outputs such as art, music, and literature.
Examples of Generative AI Applications:
- Art and Design: AI generates unique artworks and design concepts.
- Text Generation: AI writes articles, stories, and even code.
- Music Composition: AI creates original music tracks.
Key Differences Between AI and Generative AI
1. Objective and Functionality:
- Traditional AI: Aims to perform tasks that require human intelligence, such as recognizing speech, translating languages, and making decisions. It excels in analyzing data and making predictions based on patterns.
- Generative AI: Focuses on creating new content. It goes beyond analyzing and predicting to actually generating new data that resembles the input data.
2. Methodology:
- Traditional AI: Often uses algorithms like decision trees, logistic regression, and neural networks to classify data, make predictions, or automate tasks.
- Generative AI: Utilizes models like Generative Adversarial Networks (GANs), Variational Autoencoders (VAEs), and transformer-based models (e.g., GPT‑3) to generate new data.
3. Applications:
- Traditional AI: Applied in areas such as fraud detection, recommendation systems, speech recognition, and autonomous vehicles.
- Generative AI: Used in creative industries, content generation, drug discovery, and designing new products.
4. Data Utilization:
- Traditional AI: Primarily uses data to train models for classification, regression, and clustering tasks.
- Generative AI: Uses data to understand underlying patterns and generate new, similar data.
Real-World Applications and Impacts
1. Creative Industries: Generative AI has revolutionized the creative process by generating art, music, and literature. For instance, tools like DALL·E create stunning images from text descriptions, while GPT‑3 can write coherent and engaging articles.
2. Healthcare: In drug discovery, Generative AI models like GANs generate potential molecular structures that could lead to new medications, speeding up the research process significantly.
3. Marketing and Advertising: Generative AI creates personalized marketing content, such as ad copy and social media posts, tailored to specific audiences, thereby enhancing engagement and conversion rates.
4. Gaming: In the gaming industry, Generative AI is used to create realistic characters, environments, and storylines, providing a more immersive experience for players.
5. Finance: Generative AI generates realistic financial data for stress testing and scenario analysis, helping financial institutions better prepare for potential market fluctuations.
Conclusion
While AI and Generative AI share the common goal of mimicking human intelligence, their approaches and applications differ significantly. Traditional AI focuses on performing tasks that require human-like intelligence, while Generative AI goes a step further by creating new content. Understanding these differences is crucial for leveraging the right technology to solve specific problems and drive innovation.