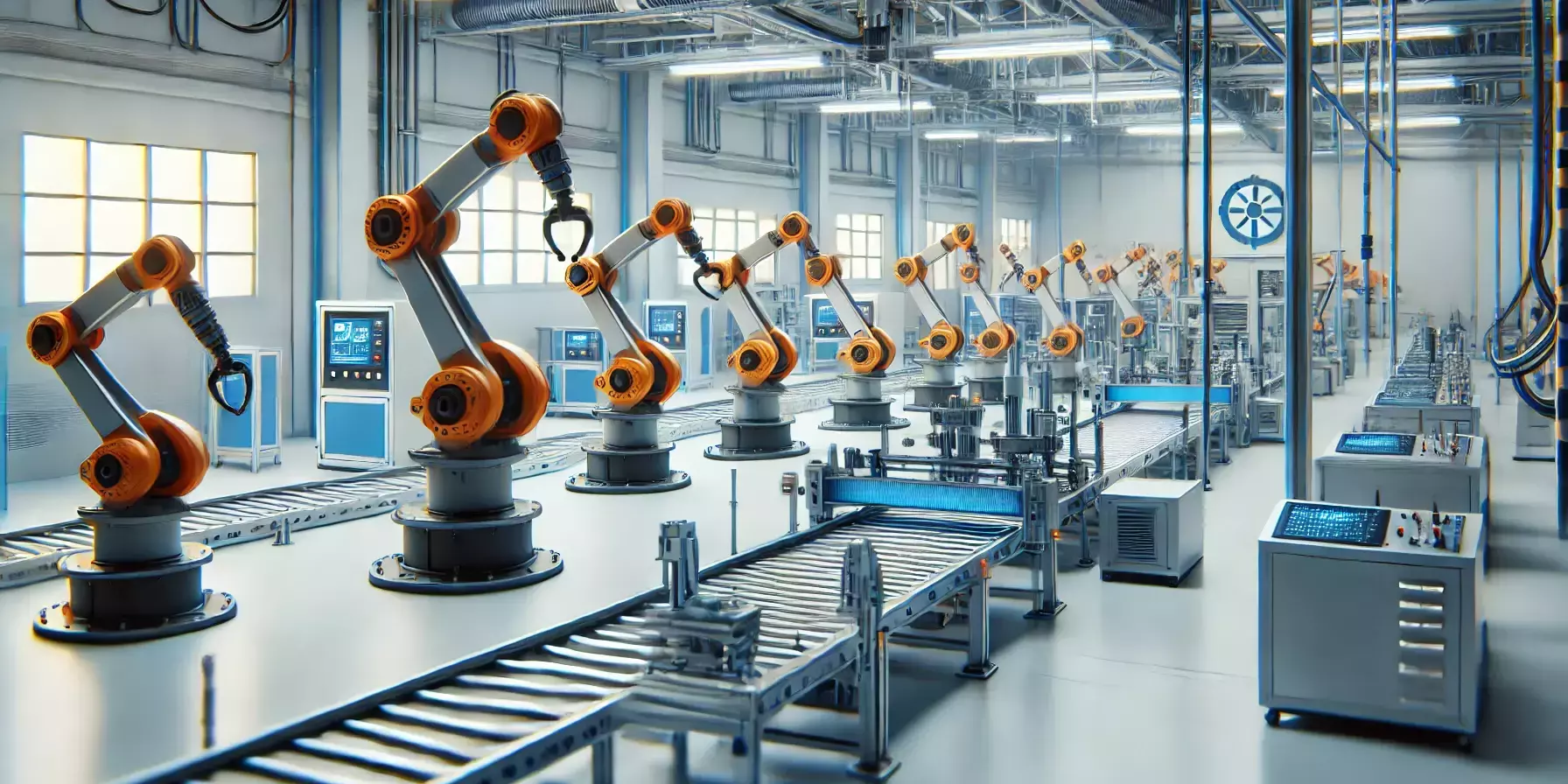
Let’s be honest: building machine learning models can feel like trying to solve a Rubik’s Cube blindfolded. You tweak hyperparameters, fiddle with feature engineering, and cross-validate until your laptop fan sounds like a jet engine. And even then, there’s no guarantee your model will perform well. Enter Automated Machine Learning, or AutoML — a suite of tools designed to streamline this process, promising to make model optimization faster, easier, and more accessible. But is it really the silver bullet it’s cracked up to be? Or are we just trading one set of headaches for another?
What Exactly Is AutoML?
At its core, AutoML is about automating the repetitive, time-consuming tasks in the machine learning pipeline. Think of it as your personal data science assistant. It can handle everything from data preprocessing and feature selection to hyperparameter tuning and model selection. Tools like Google’s AutoML, H2O.ai, and DataRobot have gained traction in recent years, offering user-friendly interfaces that let even non-experts build decent models. For seasoned data scientists, libraries like AutoKeras and TPOT provide more flexibility, allowing you to dive into the code and customize the automation process.
But here’s the kicker: AutoML isn’t just about saving time. It’s about democratizing machine learning. By lowering the barrier to entry, these tools empower smaller teams and organizations to leverage AI without needing a Ph.D. in statistics. Take, for example, a small e‑commerce startup that used H2O.ai to optimize its recommendation engine. Without a dedicated data science team, they were able to deploy a model that increased sales by 15% in just a few weeks. That’s the kind of impact AutoML can have.
Real-World Wins (and Some Caveats)
AutoML has already proven its worth in several industries. In healthcare, researchers used AutoML to predict patient readmission rates, achieving accuracy comparable to manually tuned models in a fraction of the time. In finance, companies like JPMorgan have experimented with AutoML to detect fraudulent transactions, reducing false positives by 20%. These success stories are compelling, but they don’t tell the whole story.
For one, AutoML tools aren’t magic. They’re only as good as the data you feed them. Garbage in, garbage out — no amount of automation can fix a fundamentally flawed dataset. And while AutoML excels at optimizing well-defined tasks, it struggles with more nuanced problems. For instance, if you’re working on a cutting-edge research problem in natural language processing, AutoML might not have the sophistication to handle the intricacies of your domain. It’s like using a power drill to build IKEA furniture — it works great for the basics, but don’t expect it to craft a masterpiece.
The Dark Side of Automation
Of course, no discussion of AutoML would be complete without addressing the elephant in the room: ethical concerns. Automating machine learning can lead to a false sense of security. When you hand over the reins to an AutoML tool, you’re essentially outsourcing critical decisions about your model. What if the tool selects a biased feature or overlooks an important ethical consideration? For example, an AutoML system used in hiring might inadvertently prioritize gender or race as a predictive feature, perpetuating existing biases.
Then there’s the issue of transparency. Many AutoML tools operate as black boxes, making it difficult to understand how they arrived at a particular model. This lack of interpretability can be a dealbreaker in regulated industries like healthcare or finance, where explainability is non-negotiable. And let’s not forget the potential for job displacement. While AutoML can augment the work of data scientists, there’s a valid concern that it could render certain roles obsolete — or at least devalue them.
Is AutoML Worth Your Time?
So, should you invest your time in AutoML? The answer, as with most things in life, is: it depends. If you’re a data scientist drowning in repetitive tasks, AutoML can be a lifesaver. It frees you up to focus on the more creative, strategic aspects of your work — like designing experiments or interpreting results. But if you’re an AI researcher pushing the boundaries of what’s possible, AutoML might feel like training wheels. It’s not going to help you invent the next GPT‑4.
That said, even experts can benefit from AutoML in certain scenarios. For example, it’s a great way to quickly benchmark different approaches or explore new datasets. Think of it as a tool in your toolbox, not a replacement for your expertise. And if you’re just starting out in machine learning, AutoML can be a fantastic way to get your feet wet without getting overwhelmed.
The Bottom Line
AutoML is neither a panacea nor a passing fad. It’s a powerful set of tools that, when used wisely, can accelerate your workflow and democratize access to machine learning. But it’s not without its limitations and risks. As with any technology, the key is to approach it with a critical eye. Don’t let the hype blind you to its shortcomings, but don’t dismiss it outright either. After all, the goal isn’t to replace human ingenuity — it’s to amplify it.
So, the next time you’re staring down a mountain of hyperparameters, consider giving AutoML a shot. Just remember: no tool can replace the creativity, intuition, and ethical judgment that you bring to the table. And that’s something no algorithm can automate — at least, not yet.