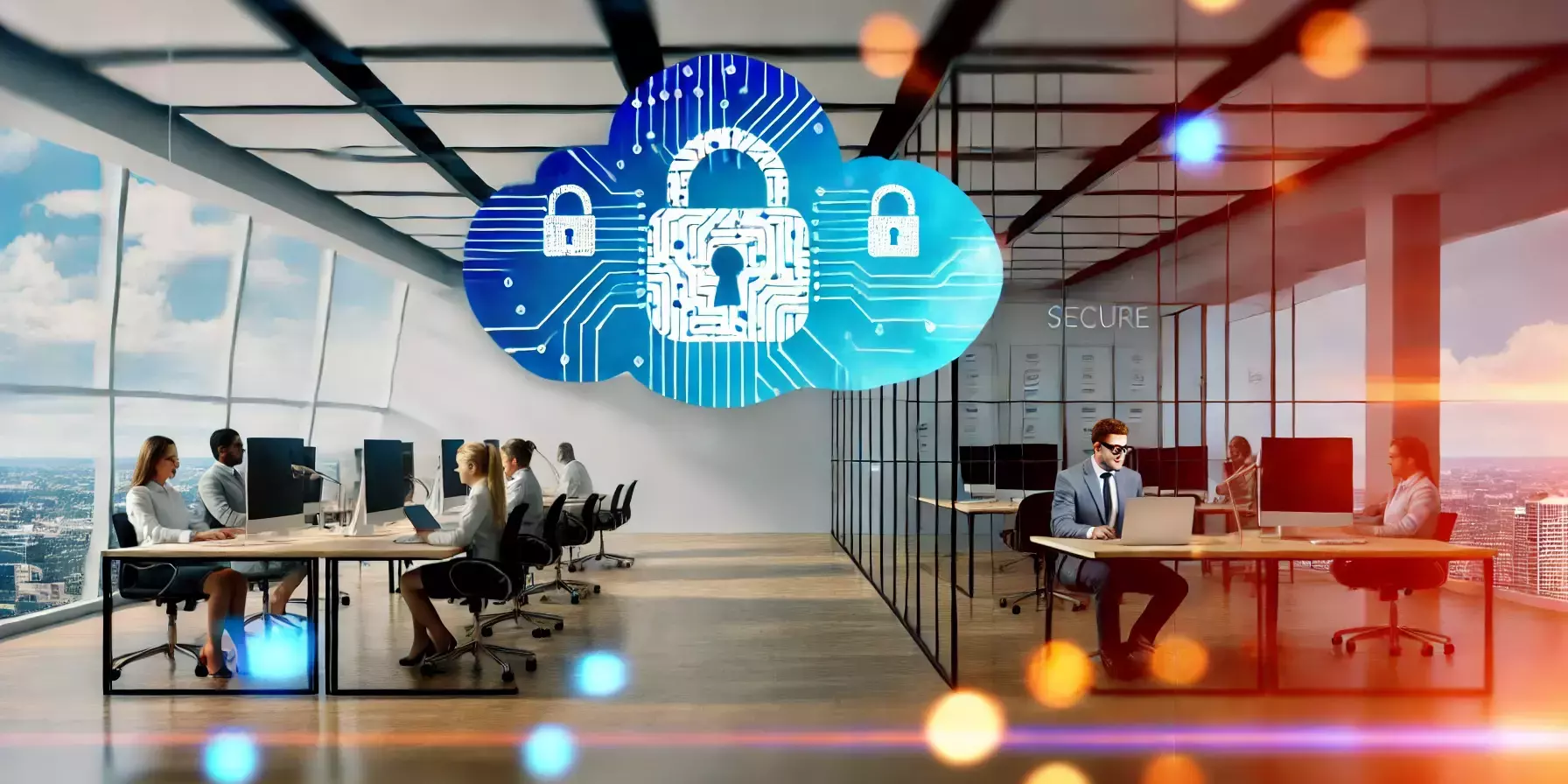
As generative AI models continue to evolve, their power to transform industries is growing in real time.. From automating creative processes to making complex decisions, generative AI is already making it’s impact in nearly every industry. However, with great power comes great responsibility. The key to harnessing this potential ethically is building strong governance frameworks and implementing effective risk management strategies. For innovators crossing the chasm from early adoption to mainstream application, this balance between innovation and ethical responsibility is crucial.
In this article, we explore how to build ethical guardrails for generative AI through robust governance and risk management frameworks, enabling businesses to innovate responsibly while minimizing risks such as bias, privacy breaches, and misuse.
Why Ethical Guardrails Matter in Generative AI
Generative AI’s ability to create new content, from text and images to entire product designs, presents unique ethical challenges. Without proper oversight, these models can unintentionally generate biased or harmful content, violate privacy laws, or be misused for malicious purposes. For businesses adopting generative AI, it is essential to ensure that these models are deployed in ways that uphold fairness, transparency, and accountability.
Key Challenges in Generative AI:
- Bias in AI models: AI models trained on biased datasets can perpetuate existing inequalities.
- Privacy risks: Generative AI often uses sensitive data, which, if mishandled, can lead to privacy breaches.
- Content misuse: Without safeguards, generative AI can be used to create misleading or harmful content, including deepfakes and misinformation(McKinsey & Company)(Stanford Cyber Policy Center).
Core Components of Generative AI Governance
Building a solid governance framework for generative AI requires a structured approach. This ensures that organizations can scale AI responsibly while managing risks.
1. Cross-Functional Governance Teams
AI governance isn’t solely a technical issue. It requires collaboration between multiple departments, including legal, ethical, technical, and business teams. A cross-functional AI governance council can oversee the development and deployment of AI systems, ensuring that various perspectives are incorporated into decision-making.
- Example: Organizations like Deloitte and IBM have created AI governance councils to manage risks across departments, ensuring that legal, technical, and ethical considerations are balanced(Deloitte United States).
By assembling a cross-functional team, businesses can develop a more holistic approach to managing the ethical and legal risks of generative AI.
2. Transparency and Accountability
Transparency is a cornerstone of effective AI governance. Generative AI models should be designed and deployed in ways that are transparent to both users and stakeholders. This includes clear documentation of how the models work, the data they rely on, and the decisions they make.
- Key Actions:
- Model Documentation: Create detailed documentation that explains how models are trained, what datasets are used, and what limitations exist.
- Regular Audits: Periodically audit AI models to ensure they are functioning as intended and adhering to ethical standards. This includes identifying potential biases and implementing corrective measures(Stanford Cyber Policy Center).
This level of transparency helps build trust in AI systems, ensuring that stakeholders understand the decisions being made and can hold organizations accountable for their outcomes.
3. Ethical AI Training and Culture
AI governance requires more than just policies — it also demands a culture of ethics. Employees, from leadership to frontline staff, must be trained on the ethical implications of AI to ensure that ethical considerations are embedded throughout the organization.
- Example: Companies like Google have introduced internal AI ethics training programs to educate employees on topics like bias, privacy, and responsible AI use(McKinsey & Company).
By fostering a culture of ethical awareness, businesses can ensure that employees across the organization are aligned on responsible AI usage.
Risk Management for Generative AI
Risk management is at the heart of AI governance. Generative AI poses unique risks that require tailored frameworks to manage effectively.
1. Bias Mitigation in AI Models
Bias is one of the most pressing risks in AI. Generative models, particularly those trained on large datasets, can inherit biases from the data they are trained on. To address this, organizations need to implement bias detection and mitigation strategies.
- Steps for Bias Mitigation:
- Diverse Data Sourcing: Ensure that training datasets are diverse and representative, reducing the likelihood of biased outcomes.
- Bias Auditing Tools: Use AI tools that detect and flag bias in models before deployment. These tools can help identify where the model might generate unfair or discriminatory content.
- Continuous Monitoring: Even after deployment, AI models should be continuously monitored for bias, ensuring they remain fair as they evolve with new data(McKinsey & Company).
2. Ensuring Data Privacy
Generative AI models rely on vast amounts of data, often including sensitive information. Managing data privacy and ensuring compliance with regulations like GDPR is essential for mitigating risks.
- Governance Actions:
- Data Anonymization: Anonymize personal data before using it in model training to protect individual privacy.
- Data Usage Policies: Establish clear policies on how data is collected, stored, and used in AI models to ensure compliance with privacy regulations(Stanford Cyber Policy Center).
Proper data governance ensures that generative AI models comply with legal standards while protecting the privacy of individuals.
3. Model Validation and Testing
Before deploying generative AI models, rigorous validation and testing are necessary to ensure they behave as expected and do not produce harmful or unintended results.
- Key Testing Practices:
- Red Teaming: A proactive approach where a group is tasked with finding vulnerabilities or risks in the AI model by attempting to misuse or break it.
- Scenario Testing: Test the model in different real-world scenarios to understand its behavior in various contexts and mitigate any risks before deployment(Deloitte United States).
Regular validation helps identify potential risks early, allowing for adjustments to the model or its governance framework before issues arise.
Building Ethical Guardrails: Best Practices for AI Governance
Developing effective governance and risk management frameworks for generative AI involves several best practices that can ensure ethical and responsible AI deployment.
- Define Clear Accountability: Assign clear responsibility for AI governance within the organization, such as appointing a Chief AI Officer to oversee AI risk management and governance policies.
- Adopt a Multi-Layered Governance Model: Implement governance structures at multiple levels, involving technical, legal, and ethical experts to create a well-rounded framework.
- Continuous Improvement: Governance frameworks should evolve with the technology. Establish a system for continuous feedback and improvement based on real-world use cases and emerging risks(McKinsey & Company)(Stanford Cyber Policy Center).
Conclusion: Innovating Responsibly with Ethical Guardrails
For innovators, the future of generative AI is filled with exciting opportunities, but those opportunities must be pursued responsibly. By building ethical guardrails through robust governance and risk management frameworks, organizations can unlock the full potential of generative AI while mitigating risks like bias, privacy violations, and misuse.
As generative AI continues to shape industries, organizations that prioritize transparency, accountability, and ethical responsibility will be best positioned to lead in the age of AI-driven innovation. The challenge is clear: to innovate boldly, but always with integrity.