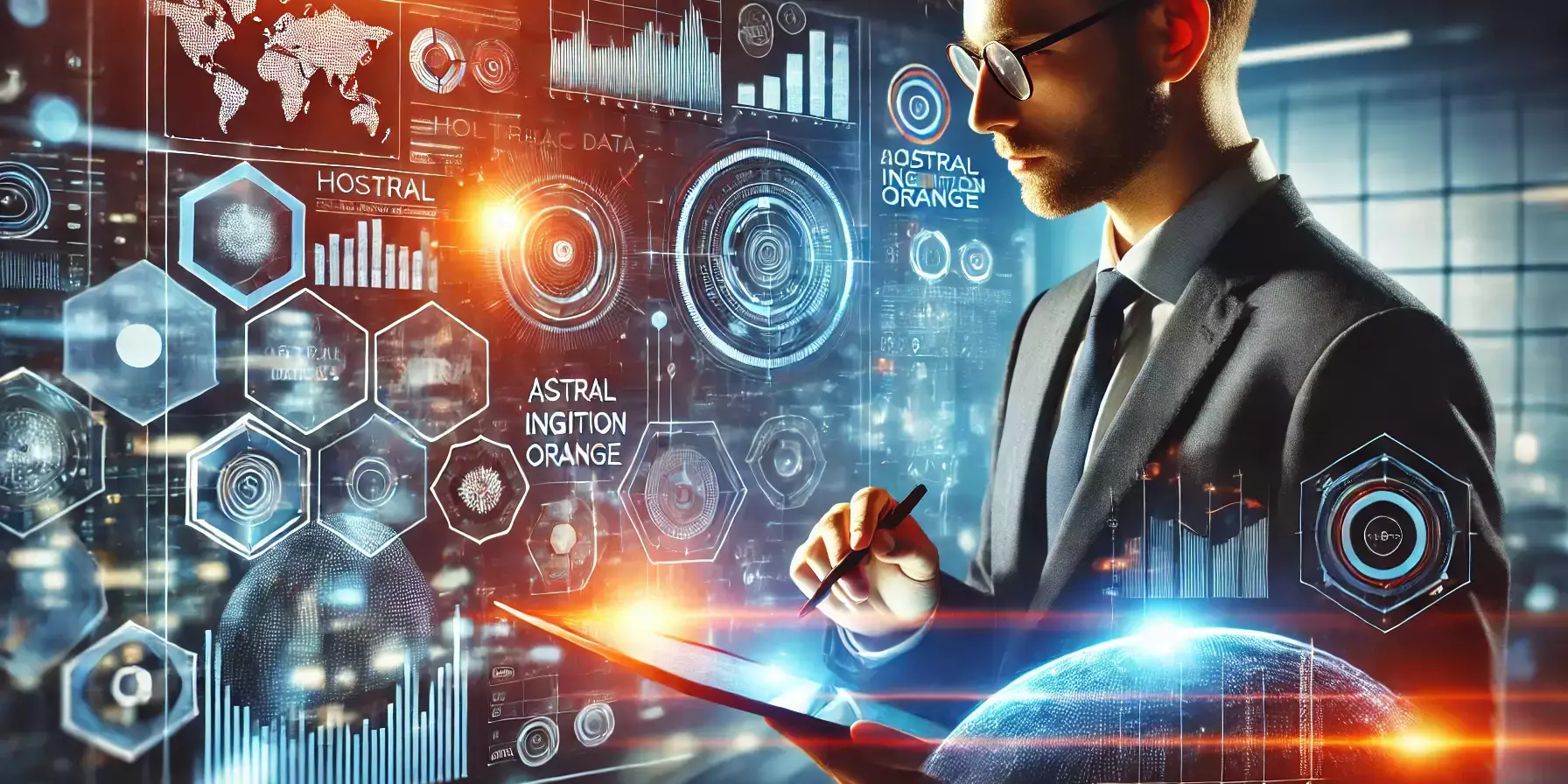
In the era of digital transformation, decision quality is a critical differentiator for businesses seeking a competitive edge. As companies strive to operate efficiently and scale effectively, the ability to make informed, timely decisions becomes paramount. This is where artificial intelligence (AI) steps in, revolutionizing business automation by empowering decision-makers with data-driven insights and real-time recommendations.
For innovators in Geoffrey Moore’s “Crossing the Chasm” model, AI-enhanced decision-making is a vital step toward mainstream adoption. By embedding AI into business automation, organizations can unlock powerful tools to improve decision quality and ensure sustainable growth.
Why Decision Quality Matters in Business Automation
In the context of business automation, decision quality refers to the accuracy, timeliness, and relevance of choices made across automated processes. High-quality decisions can reduce costs, boost productivity, and enhance customer satisfaction. However, decision quality often suffers when companies rely on outdated, manual data processes.
Challenges in Decision-Making Without AI:
- Inconsistent Data: Relying on fragmented or outdated data can lead to decisions that don’t align with current market conditions.
- Slow Decision Cycles: Without real-time insights, decision-making becomes slow, reactive, and vulnerable to error.
- Resource-Intensive Processes: Manual data analysis takes time and resources, diverting focus from strategic initiatives.
AI-powered automation provides a solution by enhancing decision quality across various functions, from supply chain management to finance and customer service.
How AI Empowers Better Decision-Making in Business Automation
AI brings a new level of precision, speed, and adaptability to decision-making, enabling organizations to make proactive, informed choices. Here’s how AI enhances decision quality across automated workflows:
1. Data Integration and Real-Time Analysis
AI excels at aggregating and analyzing data from multiple sources, providing a holistic view that improves decision-making accuracy. By analyzing real-time data, AI can identify patterns, predict outcomes, and suggest actionable insights, allowing businesses to respond proactively rather than reactively.
- Example: In retail, AI-driven tools like Google Cloud’s Retail API integrate data from inventory systems, sales records, and customer feedback to optimize pricing and stocking decisions in real time.
- Impact: Data integration ensures that decisions are based on the latest information, reducing the risk of outdated or fragmented data influencing critical choices.
2. Predictive Analytics for Proactive Decision-Making
AI leverages predictive analytics to anticipate trends, customer behavior, and market fluctuations, giving decision-makers the foresight to plan effectively. Predictive models powered by machine learning enable businesses to make proactive decisions, optimizing everything from inventory levels to resource allocation.
- Example: In logistics, companies like UPS use AI-driven predictive analytics to forecast delivery times, optimize routes, and preemptively address potential delays.
- Impact: Predictive analytics empowers businesses to make data-backed, forward-looking decisions that enhance operational efficiency and customer satisfaction.
3. Automated Risk Assessment and Mitigation
AI enhances decision quality by identifying risks and suggesting strategies for mitigation. With automated risk assessment, AI can flag potential issues, recommend preventative actions, and monitor ongoing processes to ensure adherence to regulatory standards.
- Example: Financial institutions, such as JP Morgan, use AI to automate risk analysis, identifying irregularities in financial transactions and alerting compliance teams in real time.
- Impact: Automated risk assessment reduces the potential for costly mistakes, ensuring that decisions are made with a clear understanding of associated risks.
4. Enhanced Personalization and Customer Insights
AI’s ability to analyze customer data enables companies to make decisions that are tailored to individual preferences and behaviors. By automating customer segmentation and profiling, AI allows businesses to deliver personalized experiences, improving customer engagement and loyalty.
- Example: Amazon uses AI to recommend products based on individual customer data, purchasing history, and browsing behavior, optimizing decisions on product placements and marketing strategies.
- Impact: Personalization drives customer satisfaction and loyalty by ensuring that decisions align with customer expectations and preferences.
Key Benefits of AI-Enhanced Decision Quality
For organizations that implement AI-driven decision-making, the advantages extend far beyond simple process automation. Here are the core benefits of AI-enhanced decision quality:
1. Increased Agility and Responsiveness
AI allows organizations to make rapid adjustments based on real-time data, making them more agile and responsive to changes in the market.
- Impact: Businesses can quickly adapt to new trends, competitor actions, or market demands, maintaining a competitive advantage.
2. Improved Resource Allocation
With AI, companies can make more informed decisions about how to allocate resources, reducing waste and optimizing productivity.
- Impact: Efficient resource allocation translates to cost savings and improved ROI, as businesses invest in initiatives that yield the highest returns.
3. Enhanced Accuracy and Reduced Errors
By reducing reliance on manual data entry and analysis, AI minimizes the risk of human error, leading to more accurate and reliable decisions.
- Impact: Enhanced accuracy boosts operational efficiency, ensuring that decisions are based on correct data and sound analysis.
4. Greater Compliance and Risk Management
AI’s ability to monitor compliance and assess risks ensures that decisions meet regulatory standards, reducing the likelihood of legal or financial repercussions.
- Impact: Improved compliance management safeguards business reputation and reduces the risk of costly penalties.
Real-World Applications of AI in Decision-Making Automation
AI-driven decision quality is being embraced across industries, transforming business processes and outcomes. Here are a few real-world applications:
1. Manufacturing: Optimizing Production with AI-Driven Decisions
- Use Case: Manufacturers use AI to adjust production schedules based on demand forecasts and supply chain data, optimizing output and minimizing costs.
- Example: Siemens employs AI in its factories to optimize production lines, enhancing efficiency and reducing waste.
2. Finance: Improving Investment Decisions with Predictive Analytics
- Use Case: Financial institutions leverage AI to predict stock performance, assess portfolio risk, and optimize asset allocation, improving investment outcomes.
- Example: BlackRock uses AI to drive investment decisions in its Aladdin platform, helping portfolio managers make data-driven investment choices.
3. Healthcare: Enhancing Treatment Plans with AI Decision Support
- Use Case: Healthcare providers use AI to analyze patient data, suggest treatment options, and optimize resource allocation based on patient needs and hospital capacity.
- Example: Mayo Clinic leverages AI to assist doctors in diagnosing diseases and recommending personalized treatments.
Best Practices for Implementing AI-Powered Decision Automation
Implementing AI to enhance decision quality requires a strategic approach. Here are some best practices to ensure successful adoption:
- Prioritize Data Quality: Accurate decisions depend on clean, comprehensive data. Invest in data governance and ensure that AI models are trained on reliable, up-to-date information.
- Start with High-Impact Use Cases: Identify decision points within business processes where AI can have the most significant impact, such as risk assessment, resource allocation, or customer personalization.
- Invest in Training and Change Management: Prepare your team to work alongside AI tools. Provide training on how to interpret AI-generated insights and integrate them into decision-making processes.
- Continuously Monitor and Improve: AI models learn and improve over time. Regularly assess their performance and make adjustments to optimize decision accuracy and relevance.
Conclusion: A Future of Smarter Decisions with AI
For innovators looking to cross the chasm into mainstream adoption, AI-driven decision-making in business automation offers a powerful tool to enhance decision quality, efficiency, and adaptability. By embedding AI into workflows, businesses can make data-driven, real-time choices that empower growth, minimize risk, and optimize resources.
In a world where every decision counts, AI is transforming business automation, driving better choices, and shaping a future where businesses can operate smarter and faster. For those ready to embrace AI, the possibilities for enhanced decision quality are endless — and the competitive advantage is within reach.