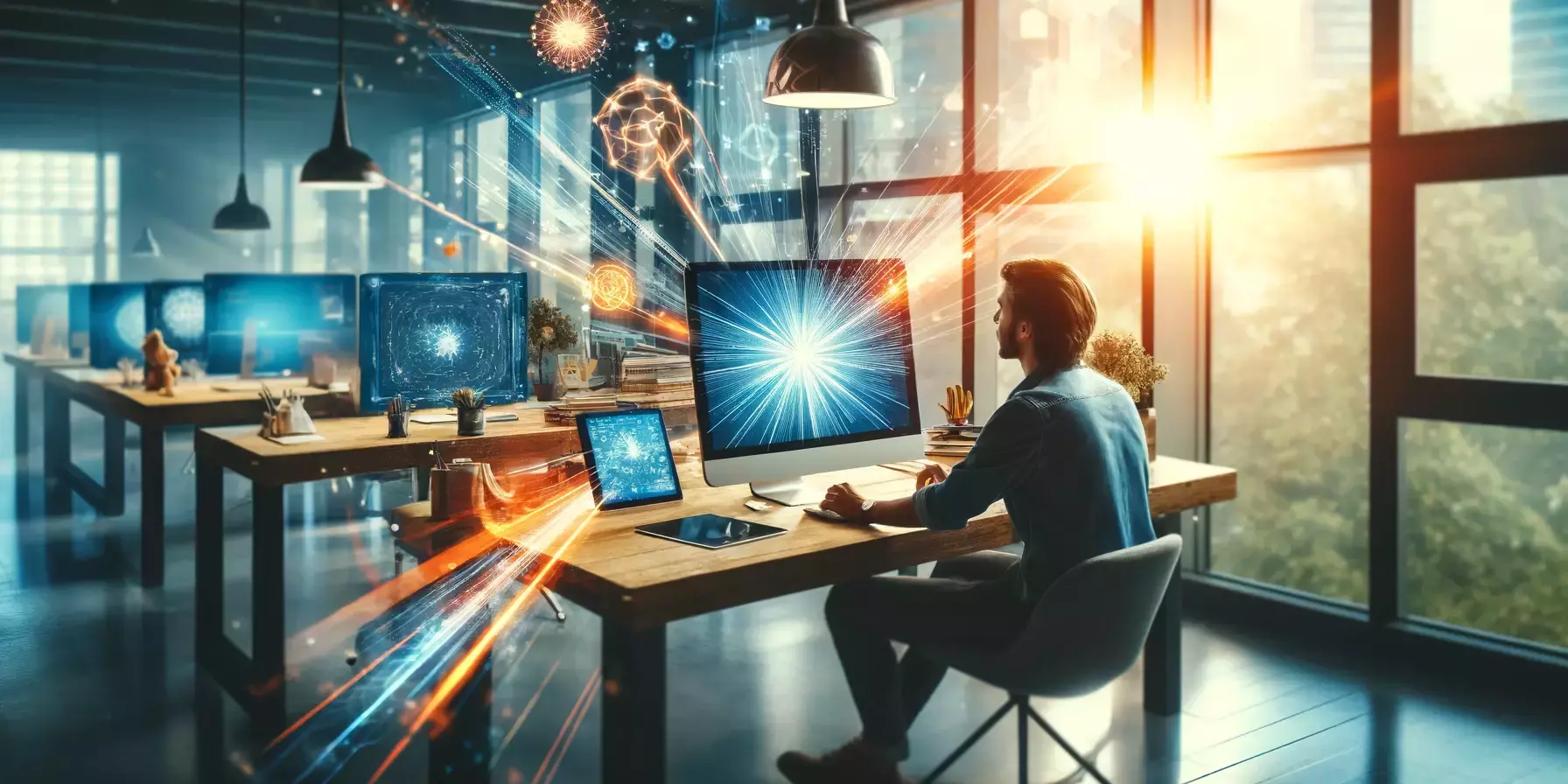
AI is revolutionizing the way work is done in the professional world in nearly ever industry, but its power comes with great responsibility. Innovators, at the forefront of this transformation, must address key ethical challenges such as fairness, bias, and transparency to ensure that AI systems are just, equitable, and trustworthy. These issues are especially pertinent when scaling AI beyond pilot projects into full operational deployment.
Understanding Ethical AI
Ethics in AI is about more than just preventing harm; it’s about creating systems that foster trust, inclusivity, and transparency. For innovators, it’s essential to ensure that AI doesn’t perpetuate existing inequalities or introduce new ones. Ethical AI frameworks help organizations navigate the complexities of fairness and transparency while maintaining their competitive edge.
Key Ethical Challenges in AI Transformation:
- Bias in AI Algorithms: AI models can inadvertently learn biases from the data they are trained on, leading to unfair treatment of certain groups.
- Lack of Transparency (Black Box AI): Some AI systems operate in ways that are difficult to interpret, making it challenging to understand how decisions are made.
- Accountability and Governance: As AI becomes more autonomous, determining who is responsible for its decisions becomes crucial.
1. Addressing Bias in AI: Striving for Fairness
One of the most significant ethical challenges in AI is ensuring fairness. AI systems, especially those driven by machine learning, can reflect and even amplify the biases present in the data they are trained on. This can lead to discriminatory outcomes in hiring, lending, law enforcement, and more.
Sources of Bias:
- Data Bias: If historical data used to train an AI model reflects societal biases (e.g., gender or racial discrimination), the model may perpetuate these biases in its outputs.
- Algorithmic Bias: Even if the data is unbiased, certain algorithms can still produce biased results due to how they weigh different variables or interpret patterns.
Solutions:
- Diverse Data Sets: Ensure that your training data represents diverse populations and scenarios. Regularly audit the data to identify and mitigate potential biases.
- Bias Detection Tools: Use AI tools that can detect and flag bias during the model development process. These tools can help ensure that algorithms treat all individuals and groups fairly.
- Cross-Functional Teams: Involve experts from diverse backgrounds, including ethicists, social scientists, and data scientists, to provide a holistic view on potential biases in AI systems.
2. Increasing Transparency: Opening the AI Black Box
AI, particularly deep learning systems, can be difficult to interpret — a phenomenon often referred to as the “black box” problem. When decisions are made by opaque systems, it’s hard to explain why certain outcomes occurred, creating a lack of accountability and trust.
Why Transparency Matters:
- User Trust: If users can’t understand why an AI system made a certain decision, they are less likely to trust it, which can harm adoption.
- Regulatory Compliance: Many industries are subject to strict regulations regarding transparency, particularly when it comes to decisions that affect individuals, such as credit scoring or healthcare.
Solutions:
- Explainable AI (XAI): Focus on developing explainable AI models that allow stakeholders to understand and trust the decision-making process. XAI provides insights into how and why decisions are made, enabling users to question and improve models as necessary.
- Clear Communication: Innovators should prioritize clarity in communicating how AI models work. Documentation, visual aids, and user-friendly interfaces can help make AI systems more accessible and transparent.
3. Accountability and Governance: Who is Responsible?
As AI systems become more autonomous, the question of accountability becomes increasingly important. Who is responsible when an AI system makes a mistake or produces harmful outcomes? Without clear governance structures, accountability can become murky, leading to potential legal and ethical issues.
Accountability Challenges:
- Autonomous Systems: When AI systems operate without human oversight, it can be difficult to pinpoint who is responsible for the system’s actions — whether it’s the developers, the organization using the AI, or the AI itself.
- Regulation Gaps: AI is evolving faster than regulation in many industries, leading to a lack of clear legal frameworks for addressing ethical concerns.
Solutions:
- AI Governance Frameworks: Implement governance frameworks that clearly define roles, responsibilities, and accountability for AI outcomes. These frameworks should outline who is responsible for monitoring AI systems, addressing errors, and ensuring compliance with ethical standards.
- Human Oversight: While AI can automate many tasks, human oversight remains critical for making complex decisions that involve ethical considerations. Ensure that AI systems are designed to involve human intervention where necessary, particularly in high-stakes scenarios.
4. Ethical AI by Design: Embedding Ethics into AI Development
For innovators leading the charge in AI transformation, embedding ethics into the design of AI systems is critical. Ethical AI by design means building systems with fairness, transparency, and accountability in mind from the outset, rather than retrofitting ethical considerations after deployment.
Key Principles for Ethical AI by Design:
- Inclusive Design: Involve a diverse range of stakeholders in the design and development of AI systems to ensure that multiple perspectives are considered, reducing the risk of bias.
- Proactive Audits: Conduct regular ethical audits of AI systems to identify potential risks and biases early in the development process. These audits should be continuous, not one-time events.
- Ethical Guidelines: Develop clear ethical guidelines that outline how AI systems should be used, including boundaries for their application and scenarios where human intervention is required.
Conclusion: Innovating Responsibly with Ethical AI
As innovators, we are all in a unique position to lead AI transformation ethically and responsibly. Ensuring fairness and transparency is not just about avoiding negative outcomes; it’s about building trust and fostering long-term success in an AI-driven world. By addressing biases, increasing transparency, and establishing strong governance frameworks, you can create AI systems that empower people, enhance customer experiences, and drive innovation — while staying true to ethical principles.
The future of AI is bright, and with a focus on fairness and transparency, you can be a leader in shaping an equitable and trusted AI landscape.