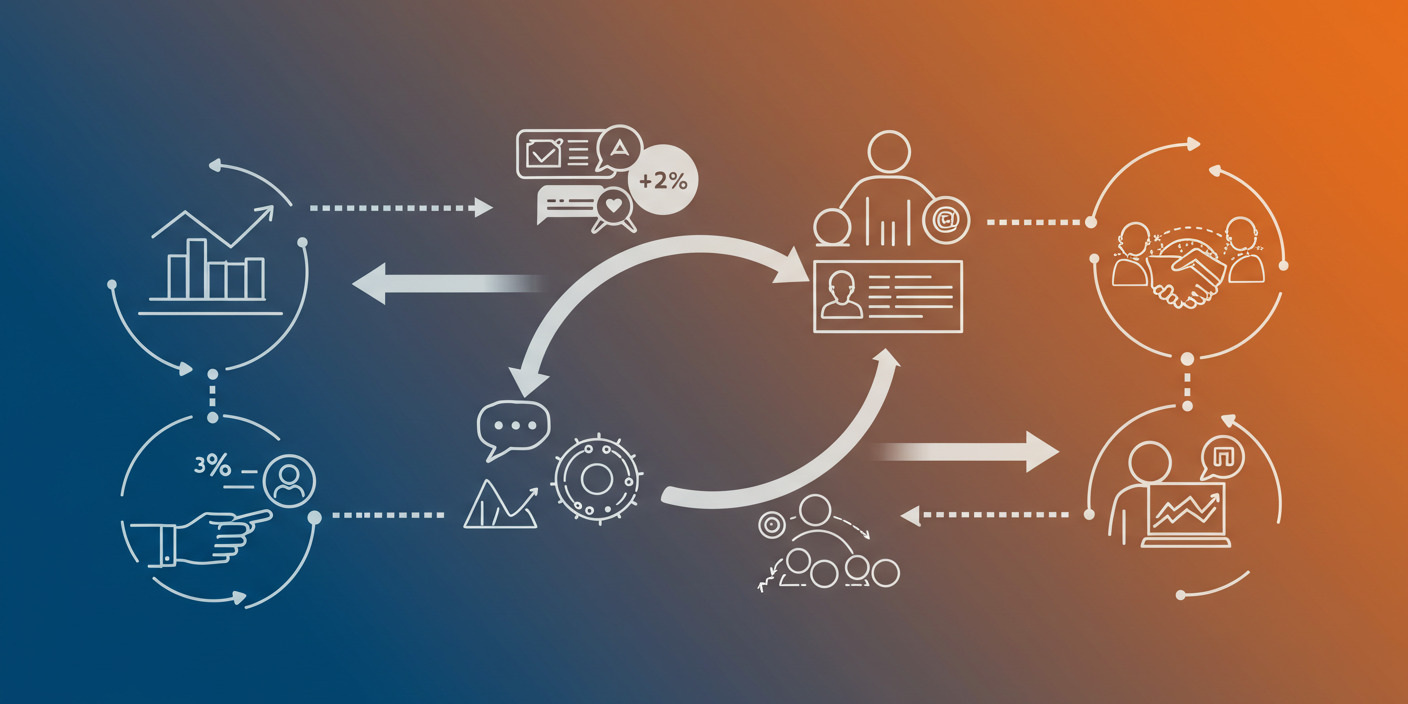
Customer journeys used to be simple. A potential buyer would see an ad, visit a store, speak with a salesperson, and maybe — if everything lined up — make a purchase. Now? That same customer might interact with your brand across ten platforms before they ever open their wallet.
Understanding that journey isn’t just important. It’s everything. And the tools we used to rely on — Excel dashboards, CRM filters, one-size-fits-all segments — simply can’t keep up.
This is where AI steps in. Not to replace the marketer or analyst, but to give them something they’ve never really had before: a continuously learning map of how, where, and why customers are moving toward — or away from — your product.
Let’s take a deeper look at the AI techniques making this possible, and why early adopters are getting ahead by embracing them.
The Customer Journey: More Fractal Than Funnel
If you’re still looking at customer journeys as funnels — top, middle, bottom — you’re missing the point. Journeys today are fragmented, recursive, and highly personalized. Customers jump from mobile to desktop to social to email, from awareness to decision and back again.
Traditional tools flatten this complexity. AI, on the other hand, can embrace it.
So, What Does AI Actually Do Differently?
AI doesn’t just collect more data. It finds relationships between signals you might not even think to compare. And it does this in real time, learning and refining as new data comes in. Here are some of the techniques powering that shift:
1. Behavioral Clustering (Unsupervised Learning)
Instead of grouping customers by demographics or purchase history, AI can segment based on behavior — site interactions, content preferences, time between purchases, and more.
Why it matters: You’re no longer marketing to “millennial women” or “enterprise buyers.” You’re marketing to behavioral patterns that transcend labels.
Used by: Streaming services like Netflix and Spotify to recommend content, e‑commerce platforms like Shopify to personalize storefronts.
2. Sequence Modeling (Recurrent Neural Networks & Transformers)
Ever wonder what a customer is likely to do next? AI models trained on behavioral sequences can predict future actions based on historical paths — like which users who added a product to cart are most likely to return and buy in the next 48 hours.
Why it matters: Timing is everything. Knowing when to reach out — or hold back — can be the difference between conversion and churn.
Used by: Travel and ticketing sites to manage offers based on booking behaviors; SaaS platforms to drive timely upsells.
3. Propensity Modeling
This technique calculates the likelihood of a given customer taking a specific action, like signing up for a webinar or clicking an ad.
Why it matters: Rather than blanket campaigns, you can target those most likely to act — improving ROI and reducing fatigue.
Used by: B2B marketers for lead scoring; retailers for offer targeting.
4. Path Analysis & Attribution Modeling
AI can analyze not just the steps a customer took, but the weight of each one in leading to conversion. It helps answer the hard question: What actually made a difference?
Why it matters: You can stop giving full credit to last-click conversions and start investing in what moves the needle upstream.
Used by: Media companies, DTC brands, and anyone juggling multi-channel marketing.
5. Anomaly Detection
This might sound like a back-office tool, but it’s a powerful tactic in customer journey mapping. Anomalies — like a sudden drop in conversions after a site redesign — can be flagged instantly by AI without setting up a hundred filters manually.
Why it matters: You don’t have to wait for your quarterly review to notice something’s off.
Used by: Retailers during high-stakes shopping seasons, SaaS companies after new feature launches.
Why Early Adopters Are Doubling Down on Journey Mapping AI
Companies that integrate AI into customer journey analysis aren’t just optimizing performance — they’re unlocking new strategic questions:
-
What paths convert fastest — and which ones never convert at all?
-
Which high-LTV users are slipping away without ever contacting support?
-
What seemingly low-value interactions are silently powering loyalty?
These aren’t things you uncover with surface-level dashboards. They require systems that can see relationships in data we wouldn’t think to connect — and update those insights dynamically.
But It’s Not Plug-and-Play
There’s a reason not every company is doing this yet. AI journey mapping comes with its challenges:
-
Data fragmentation: Your web data is in one system, email data in another, customer feedback in a third. Integration takes work.
-
Interpretability: Just because a model says X leads to Y doesn’t mean your team understands why — or trusts it.
-
Ethical oversight: You’re analyzing human behavior, sometimes deeply personal. Respect, transparency, and compliance aren’t optional.
Still, these are solvable problems — and the companies solving them now are building a serious moat.
Final Thoughts: You Can’t Optimize What You Can’t See
Customer behavior is getting more complex, not less. That means the teams that see clearly will win. AI isn’t here to replace your marketing instincts or creative spark. But it is here to help you see things — journey patterns, drop-off points, key moments — that used to be invisible.
Early adopters aren’t just adding new tools. They’re building a new mindset: one that expects data to evolve, expects patterns to shift, and knows that real insight comes from embracing the complexity of the journey — not trying to flatten it.
So the next time someone hands you a “customer journey map” built on averages and assumptions, ask a simple question:
What are we not seeing?
That’s where AI starts to do its best work.