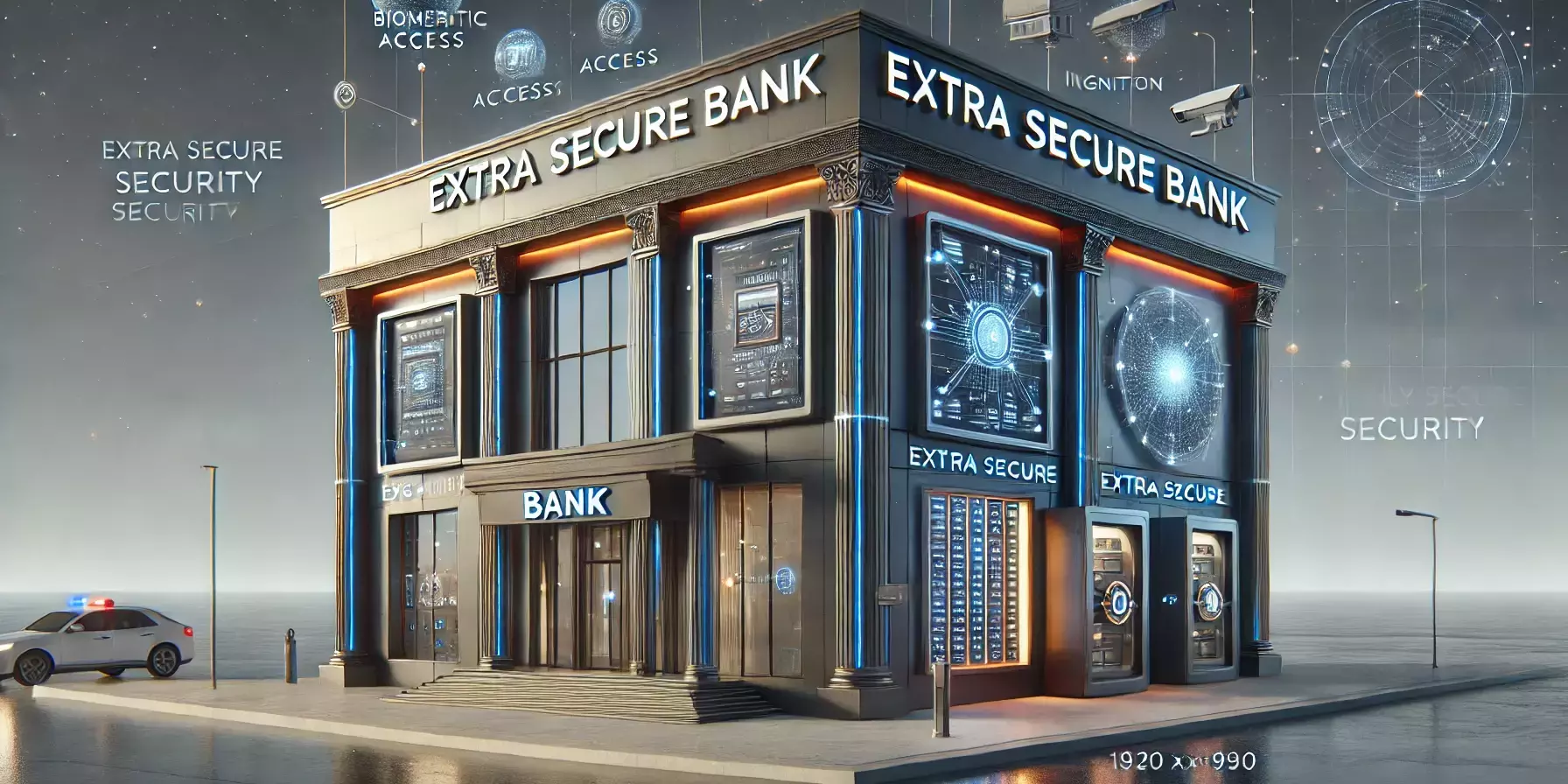
As businesses increasingly adopt multi-cloud environments to optimize scalability, resilience, and flexibility, ensuring robust security across these platforms has become a top priority. With the complexity of managing security across multiple cloud providers, traditional methods are no longer sufficient. Enter AI-driven security solutions, which bring agility, intelligence, and precision to safeguarding multi-cloud environments.
For early adopters, understanding the future trends in AI-driven security is essential for staying ahead of threats while maximizing the benefits of multi-cloud strategies. This article explores emerging trends and actionable strategies to future-proof your cloud security.
Why AI-Driven Security is Essential for Multi-Cloud Environments
Multi-cloud environments involve using multiple cloud platforms, such as AWS, Google Cloud, and Microsoft Azure, to store data, run applications, and manage operations. While this approach offers flexibility and redundancy, it also introduces challenges, including:
- Diverse security protocols: Each cloud provider has its own set of rules and tools.
- Increased attack surface: More endpoints mean more opportunities for cyberattacks.
- Manual monitoring limitations: Traditional methods struggle to keep up with the speed and scale of multi-cloud operations.
AI addresses these challenges by providing real-time threat detection, automated responses, and predictive analytics, transforming how businesses approach security.
Emerging Trends in AI-Driven Security for Multi-Cloud Environments
1. Predictive Threat Intelligence
AI-driven systems are becoming increasingly adept at predicting cyber threats before they occur. By analyzing vast amounts of data from network traffic, system logs, and external threat feeds, AI can identify patterns that indicate potential attacks.
- Example: Predictive models can detect unusual login behaviors or anomalous data transfers, flagging potential breaches before they escalate.
- Impact:
- Enhanced ability to proactively address vulnerabilities.
- Reduced downtime caused by security incidents.
- Improved trust among stakeholders.
2. Zero Trust Security with AI Enforcement
The Zero Trust model assumes that no user or device should be trusted by default, even within the network perimeter. AI enhances Zero Trust by continuously monitoring and validating users, devices, and applications in real time.
- Example: AI algorithms assess user behavior and device health to enforce adaptive access policies dynamically.
- Impact:
- Strengthened defense against insider threats.
- Real-time adjustments to access permissions based on risk levels.
- Seamless integration with existing multi-cloud architectures.
3. AI-Powered Anomaly Detection
AI excels at identifying anomalies that might escape traditional rule-based systems. In multi-cloud environments, where traffic and interactions are highly dynamic, anomaly detection becomes even more critical.
- Example: AI can flag abnormal data transfer rates between cloud platforms, signaling potential data exfiltration attempts.
- Impact:
- Faster detection of subtle threats.
- Reduced false positives compared to manual monitoring.
- Greater confidence in identifying previously unknown attack vectors.
4. Intelligent Data Encryption and Privacy Preservation
AI is revolutionizing data encryption by dynamically adapting encryption levels based on sensitivity and context. It also ensures that data privacy regulations are met across different jurisdictions.
- Example: AI systems automatically encrypt data being transferred between cloud providers based on compliance requirements.
- Impact:
- Enhanced protection for sensitive information.
- Simplified compliance with regulations like GDPR and CCPA.
- Reduced human error in managing encryption protocols.
5. Unified Security Management Across Clouds
Managing security across multiple cloud providers can be fragmented and cumbersome. AI tools are evolving to provide unified security dashboards that give businesses a comprehensive view of their multi-cloud environment.
- Example: AI-powered platforms consolidate security metrics, alerts, and compliance statuses from AWS, Azure, and Google Cloud into a single interface.
- Impact:
- Streamlined monitoring and reporting.
- Faster resolution of security incidents.
- Greater transparency and control over multi-cloud environments.
6. Edge AI for Real-Time Decision-Making
With the rise of edge computing, AI is moving closer to where data is generated. This trend enables real-time security decisions without relying on centralized cloud systems, reducing latency and enhancing responsiveness.
- Example: AI algorithms at the edge can instantly block unauthorized access attempts or isolate infected devices before they connect to the broader network.
- Impact:
- Faster response times to threats.
- Reduced bandwidth usage for centralized processing.
- Enhanced security for IoT and edge devices.
Key Considerations for Adopting AI-Driven Security
1. Data Quality and Availability
- AI models rely on high-quality, comprehensive data to deliver accurate results.
- Action Step: Conduct regular data audits to ensure security data from all cloud providers is integrated and clean.
2. Scalability
- As your multi-cloud environment grows, your security solutions must scale with it.
- Action Step: Choose AI tools that are cloud-agnostic and capable of handling large-scale environments.
3. Vendor Collaboration
- AI security tools often require collaboration with multiple cloud providers.
- Action Step: Work closely with vendors to ensure compatibility and seamless integration across platforms.
4. Team Training and Change Management
- AI adoption requires new skills and processes within security teams.
- Action Step: Invest in training programs to upskill teams and foster a culture of innovation.
The Future of AI-Driven Security: What to Expect
Looking ahead, AI-driven security solutions will continue to evolve, introducing new capabilities and transforming how businesses protect their multi-cloud environments. Key trends include:
- Hyperautomation in Security: Combining AI with automation to create self-healing systems that respond to threats autonomously.
- Federated Learning for Global Threat Intelligence: Using federated AI models to share threat insights across organizations without compromising data privacy.
- Quantum-Resistant AI Algorithms: Preparing for the next generation of cybersecurity threats posed by quantum computing.
Conclusion: Embracing AI for a Secure Multi-Cloud Future
For early adopters, AI-driven security represents an opportunity to not just keep pace with evolving threats but to stay ahead of them. By leveraging predictive analytics, Zero Trust frameworks, and intelligent anomaly detection, businesses can transform their multi-cloud environments into secure, resilient ecosystems.
The future of AI in security is bright, filled with potential to simplify complexity, reduce risks, and build trust in a hyperconnected world. For those ready to lead the charge, the time to embrace AI-driven security is now. The result? A safer, smarter, and more agile multi-cloud infrastructure.