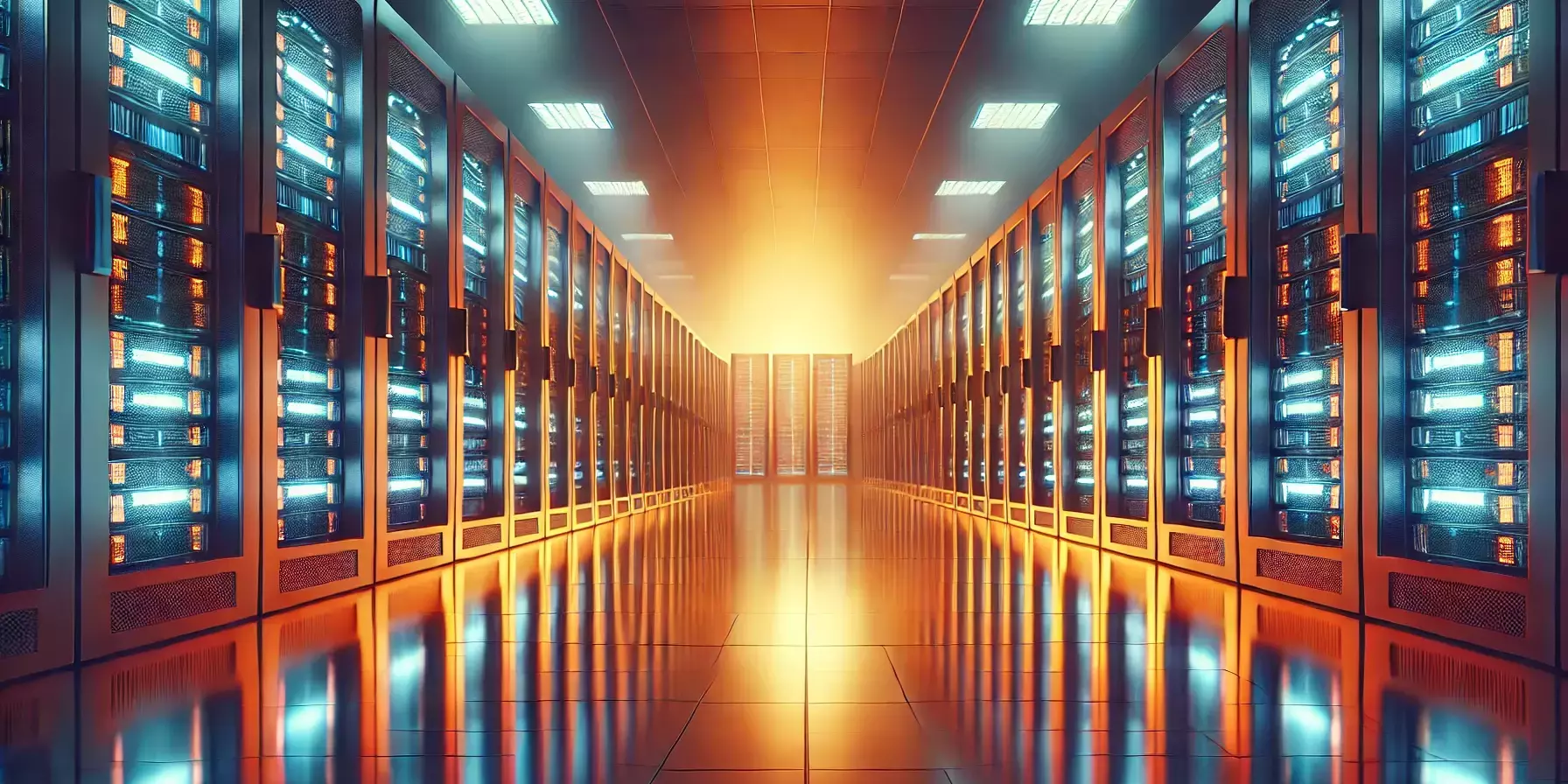
The AI revolution is here, and innovators are at the forefront of this transformative wave, pushing boundaries and redefining industries. But as exciting as AI-driven business transformation can be, it comes with its own set of challenges, particularly around data quality. Data is the lifeblood of AI, and any issues with its quality can severely impact the success of AI initiatives. In this post, we’ll explore the common data quality challenges innovators face in AI implementation and provide practical solutions to overcome them.
The Importance of Data Quality in AI
AI systems rely on vast amounts of data to learn, make decisions, and generate insights. However, the effectiveness of these systems is directly proportional to the quality of the data they are fed. Poor data quality can lead to inaccurate models, biased outcomes, and ultimately, the failure of AI projects. For innovators who are leading the charge in AI-driven business transformation, ensuring data quality is not just a technical requirement; it’s a strategic imperative.
Common Data Quality Challenges in AI Implementation
1. Data Silos: Fragmented and Isolated Data Sources
One of the most pervasive challenges in AI implementation is dealing with data silos. In many organizations, data is stored across various departments and systems, leading to fragmentation. This isolation of data makes it difficult to access a holistic view, which is crucial for training AI models effectively.
- Impact: AI systems trained on incomplete or fragmented data sets are likely to produce skewed results that do not reflect the true state of the business.
- Solution: Implementing data integration platforms that can consolidate data from various sources is essential. Additionally, fostering a culture of data sharing across departments can help break down these silos.
2. Inconsistent Data: Variability in Data Formats and Standards
Data inconsistency is another major hurdle in AI implementation. When data comes from multiple sources, it often varies in format, structure, and standards. This variability can lead to significant challenges in data processing and model training.
- Impact: Inconsistent data can confuse AI models, leading to inaccurate predictions and insights.
- Solution: Standardizing data formats and establishing clear data governance policies can mitigate this issue. Innovators should also invest in data cleaning and preprocessing tools that can automate the standardization process.
3. Data Accuracy: The Need for Reliable and Correct Data
The accuracy of data is fundamental to the success of any AI initiative. However, inaccuracies can creep in due to human error, outdated information, or system glitches. These inaccuracies can be disastrous for AI models, leading to flawed insights and decisions.
- Impact: Inaccurate data can result in poor decision-making, ultimately harming business outcomes.
- Solution: Implement robust data validation processes to ensure that the data fed into AI systems is accurate and up-to-date. Regular audits and the use of machine learning models to detect anomalies in data can also help maintain accuracy.
4. Data Completeness: Ensuring All Relevant Data is Captured
Incomplete data is another significant challenge. AI models need comprehensive data to make accurate predictions. Missing data points can lead to biased models that fail to generalize across different scenarios.
- Impact: Incomplete data can lead to biased models, reducing their effectiveness and reliability.
- Solution: Implementing automated data collection systems can help ensure that all relevant data is captured. Additionally, filling in missing data using statistical methods or machine learning algorithms can help create more complete datasets.
5. Data Timeliness: Keeping Data Current and Relevant
In the fast-paced world of AI, outdated data can quickly become irrelevant. Timely data is crucial for making accurate predictions and staying ahead of the competition. However, ensuring that data is current and up-to-date can be challenging, especially in dynamic environments.
- Impact: Outdated data can lead to decisions that are no longer relevant, causing missed opportunities or costly mistakes.
- Solution: Implementing real-time data processing systems and setting up automated data pipelines can ensure that your data remains current. Innovators should also establish protocols for regularly updating and refreshing their data sources.
Strategic Solutions for Innovators
For innovators looking to cross the chasm and lead successful AI-driven transformations, addressing these data quality challenges is critical. Here are some strategic solutions to consider:
1. Invest in Data Governance
Data governance is the backbone of data quality. Establishing clear policies, roles, and responsibilities for data management can help ensure that data is accurate, consistent, and reliable. Innovators should advocate for data governance frameworks that align with their AI objectives and foster a culture of data excellence within their organizations.
2. Leverage AI for Data Quality Improvement
AI itself can be a powerful tool for improving data quality. Machine learning algorithms can be used to detect anomalies, predict missing values, and even standardize data formats automatically. By leveraging AI to enhance data quality, innovators can create a virtuous cycle where AI improves the very data it relies on.
3. Foster a Collaborative Data Culture
Breaking down silos and encouraging collaboration across departments is essential for data quality. Innovators should lead the charge in creating a culture where data is shared freely and responsibly across the organization. This not only improves data quality but also accelerates AI adoption by making data more accessible to AI initiatives.
Conclusion: Turning Challenges into Opportunities
While data quality challenges can be daunting, they also present a unique opportunity for innovators to differentiate themselves. By addressing these challenges head-on and implementing strategic solutions, you can ensure that your AI-driven business transformation is built on a solid foundation of high-quality data. Remember, the future of AI is bright, and with the right approach to data quality, you can lead your organization into a new era of innovation and success. The path forward may be complex, but with imagination, positivity, and an unwavering commitment to excellence, the rewards are well worth the effort.