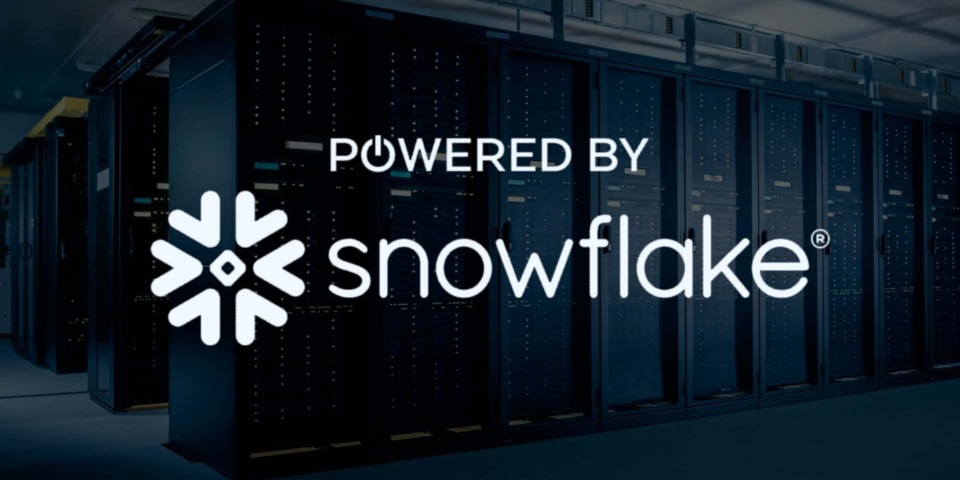
Maintaining a modern data stack that meets the needs of a growing organization is a challenge, both technically and financially. This challenge is amplified when companies grow through acquisitions, leading to duplicative data, tools, and processes. However, consolidating and rationalizing legacy systems presents an opportunity to optimize the integrated data stack, leading to a more efficient and effective system for a growing business.
Unlocking data for a leading PE firm
When a leading private equity firm entered a new tech domain, they rolled together 4 disparate companies, all under a consolidated brand. This vertically integrated the industry in a completely new way; however, the data was all stored in legacy transactional systems and disjointed data warehouses. This blocked the company’s ability to consolidate customer data, cross-sell and upsell products, and optimize applications with new enhanced data sources from new acquisitions. This also blocked consolidated analytics and business intelligence, and limited machine learning and generative AI opportunities which jeopardized our customers targeted cost and revenue synergies.
The Stellar team developed a plan to unify the data with these goals in mind:
- get to benefits quickly (cost optimization and revenue expansion)
- build for long term scalability
- ensure cloud agnostic so can move and optimize data over time
- ensure one data source of truth driving the combined business
- ensure an analytics and AI ready data stack
Benefits Quickly
After establishing a demo instance on Snowflake, we deployed our ELT tool, Fivetran, to start moving data. One of the key advantages of Snowflake is its ability to handle large volumes of data with ease, allowing us to replicate raw transactional data from the source systems without worrying about data storage and processing limitations. This provided us with a centralized data repository that could be accessed easily by other processes and products, making it easier to align our diverse data operations. We started by migrating data to our new Snowflake instance company by company. This allowed us to move quickly and in parallel with other operational priorities. This approach turned a large, unwieldy project into one that had many manageable tasks that could bring value to the customer from Day 1.
Building Scalability
Snowflake is highly scalable due to its unique architecture, which separates compute and storage layers. This allows users to easily scale up or down their compute resources independent of their data storage, making it easy to handle large amounts of data with ease. Additionally, Snowflake’s automatic scaling capabilities mean that users can easily add or remove compute resources as needed, without worrying about manual intervention or downtime. The scalable Snowflake instance allowed us to focus on the desired business outcomes for the PE firm, while ensuring the right construct of the data and schemas, pipeline and ingest data, for long term ease of use and to facilitate future acquisitions.
Cloud agnostic
One of the key advantages of Snowflake is that it is cloud agnostic, meaning that it can run on various cloud platforms such as Amazon Web Services (AWS), Microsoft Azure, and Google Cloud Platform (GCP). This flexibility allows users to choose the cloud platform that best fits their needs and switch between cloud providers as needed, without having to worry about data migration or platform lock-in. This was a particularly useful feature for our customer for both DR and for their end customer access. We could determine our customer’s cloud platform, but not their end customer’s platform. Snowflake made it easy to replicate a full copy of a customer’s data, on any cloud platform, enabling the end customer to easily amend their existing database or warehouse with the data flowing from our service and continue to leverage their cloud of choice, all seamlessly managed via Snowflake.
Analytics and AI Ready Data
When imagining the ideal data environment with our customer, we aimed to not only provide a great in-app analytics and reporting experience but also to offer a straightforward method for end customers to use a complete copy of their data natively in their own data environment and in conjunction with their own BI tools. To accomplish this, we created a branded Data Exchange for our customer. With a few simple steps, we were able to provide connection strings to the end customers, allowing them to combine our customers data with their internal and operational data, providing end customers with a unique and comprehensive data set to support more advanced analytics and generative AI solutions.
Snowflake’s unique architecture separates compute and storage layers, which allows for the rapid querying of data regardless of its size or complexity. This scalability made it easy for our customer to handle vast amounts of data, including unstructured data, and integrate it into their analytics and machine learning workflows. Additionally, Snowflake’s built-in machine learning capabilities, combined with its ability to seamlessly integrate with popular machine learning tools, allow us to perform advanced analytics and predictive modeling on top of the consolidated data warehouse. By having analytics and machine learning ready data, our customers make better data-driven decisions, gain insights into their operations, and identify opportunities for growth and optimization.
Leverage Stellar’s experience to integrate your data, provide practical machine learning solutions, and accelerate your cost and revenue synergies in merger and acquisition efforts.