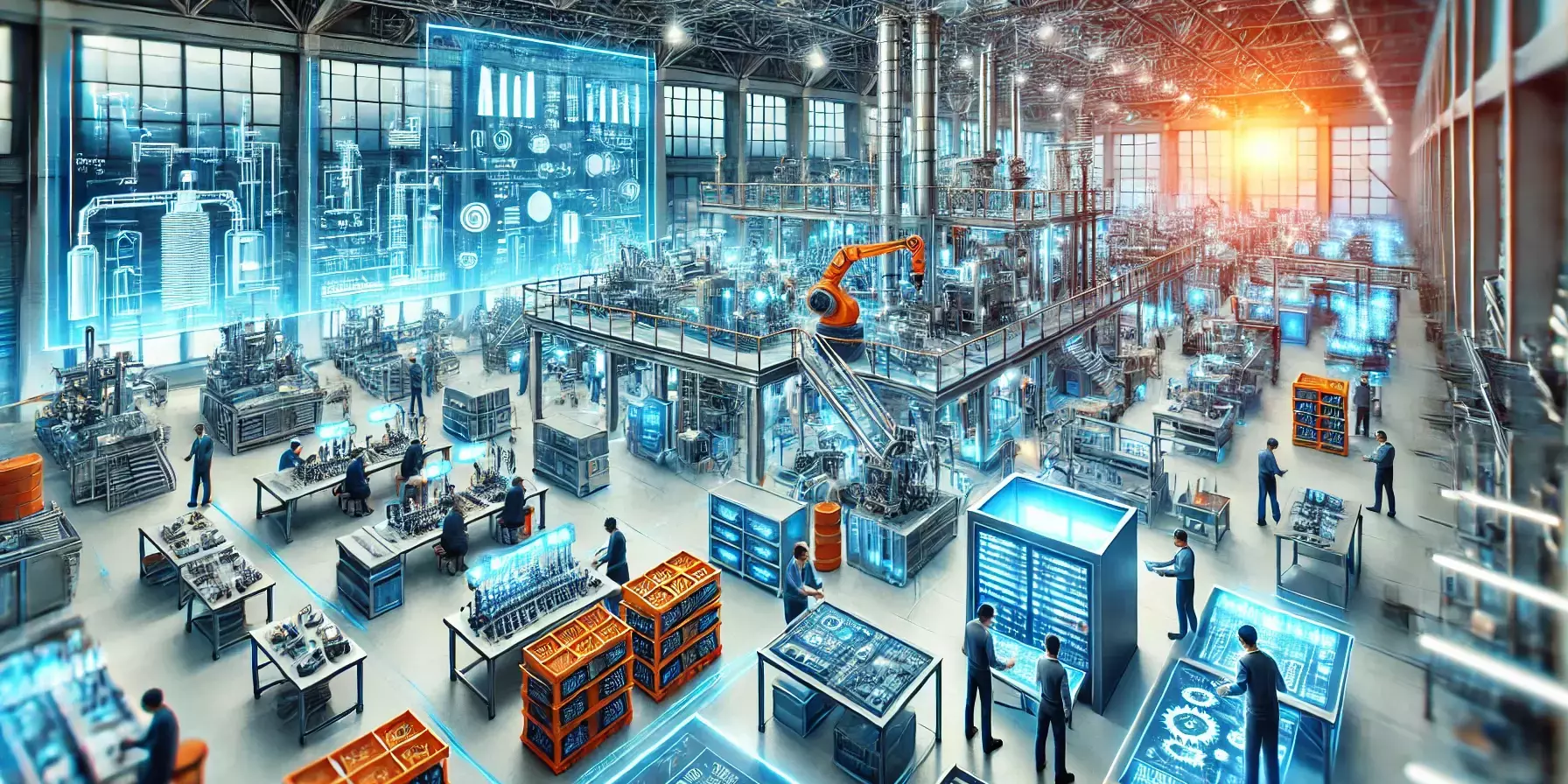
In today’s fast-paced global economy, AI-driven supply chain automation is no longer a luxury — it’s a necessity. The integration of artificial intelligence (AI) into supply chain processes is transforming how companies operate, offering efficiency gains, cost savings, and a competitive edge. However, the journey to successfully implementing AI in the supply chain is not without its challenges.
For innovators at the forefront of this transformation, understanding and overcoming these obstacles is key to reaching mainstream adoption. This guide explores common challenges in AI-driven supply chain automation and provides practical strategies to navigate them.
The Promises of AI in Supply Chain Automation
Before diving into the challenges, it’s essential to recognize the immense value AI brings to the supply chain. By automating processes and making intelligent, data-driven decisions, AI can:
- Improve demand forecasting: AI analyzes historical data and market trends to predict future demand with greater accuracy.
- Optimize inventory management: AI automates stock levels, reducing excess inventory while preventing stockouts.
- Enhance logistics and delivery: AI can plan routes, reduce transportation costs, and minimize delivery times.
- Enable real-time monitoring: AI provides visibility across the supply chain, enabling companies to react swiftly to disruptions.
Yet, the road to realizing these benefits is often filled with technical, operational, and cultural hurdles. Let’s examine the most common challenges and how businesses can overcome them.
Challenge 1: Data Integration and Quality Issues
One of the biggest challenges in AI-driven supply chain automation is the integration and quality of data. AI systems rely heavily on accurate, real-time data to function effectively, but many organizations struggle with siloed, incomplete, or outdated data sources.
Solutions:
- Data Unification and Cleansing: Start by consolidating data from disparate sources into a unified system. Use ETL (Extract, Transform, Load) processes to clean and standardize data before feeding it into AI systems.
- Cloud-Based Solutions: Cloud platforms such as AWS and Microsoft Azure offer robust tools for managing, storing, and processing data, ensuring that AI models have access to clean and up-to-date dataAgreeYa Solutions.
- Continuous Monitoring: Regularly audit your data pipelines to ensure the information flowing into your AI systems is accurate and relevant. Use AI-driven data quality tools to identify anomalies and correct errors in real time.
Challenge 2: Lack of AI Expertise
AI implementation requires specialized knowledge that may be lacking in traditional supply chain teams. Organizations often struggle to find employees with the technical expertise to develop, deploy, and manage AI systems.
Solutions:
- Partner with AI Vendors: Companies like UiPath, Blue Yonder, and IBM Watson offer AI solutions tailored to supply chain needs, helping businesses implement AI without needing deep in-house expertise.
- Upskill Your Workforce: Invest in training programs to build AI expertise within your organization. Many online platforms, such as Coursera and Udemy, offer AI and machine learning courses specifically designed for business applications.
- Cross-Functional Teams: Create cross-functional teams that combine supply chain professionals with IT and AI experts to ensure successful implementation and collaboration.
Challenge 3: Resistance to Change and Organizational Buy-In
Implementing AI in the supply chain often requires significant shifts in processes, workflows, and job roles. Resistance from employees and even leadership can slow down or derail AI initiatives.
Solutions:
- Change Management: Focus on communicating the benefits of AI clearly to all stakeholders. Highlight how automation can reduce manual tasks, improve efficiency, and allow teams to focus on strategic activities.
- Pilot Programs: Start with small, manageable AI projects that demonstrate quick wins. A successful pilot can help build confidence in AI initiatives and create momentum for broader adoption.
- Leadership Champions: Secure buy-in from senior leaders who can advocate for AI-driven supply chain automation and provide the necessary resources and support for successful implementation.
Challenge 4: Integration with Legacy Systems
Many organizations still rely on legacy systems that were not built to integrate with modern AI platforms. This can create barriers to automation, as outdated systems lack the flexibility and connectivity required for seamless AI deployment.
Solutions:
- APIs and Middleware: Use Application Programming Interfaces (APIs) and middleware to bridge the gap between legacy systems and AI platforms. These tools allow for communication between old and new systems, facilitating smoother integration.
- Incremental Modernization: Rather than replacing legacy systems entirely, adopt a phased approach. Start by upgrading the most critical parts of the system and gradually integrate AI capabilities.
- Cloud Migration: Migrate legacy systems to the cloud where AI tools can be more easily integrated. Cloud-based systems are more scalable and offer better support for AI and data analytics.
Challenge 5: Cost and ROI Justification
Implementing AI in the supply chain can require significant upfront investment, making it difficult for some businesses to justify the costs, especially if the ROI is not immediately clear.
Solutions:
- Focus on High-Impact Areas: Identify areas in the supply chain where AI can deliver the most immediate benefits, such as demand forecasting or inventory management. Prioritize projects that demonstrate clear cost savings or efficiency gains.
- Measure Success with KPIs: Establish clear Key Performance Indicators (KPIs) to measure the success of your AI initiatives. These might include improved delivery times, reduced stockouts, or lower operational costs.
- AI-as-a-Service: Consider adopting AI-as-a-Service (AIaaS) platforms, which offer AI solutions on a subscription basis. This reduces the upfront cost of AI implementation and allows companies to scale usage based on their needs.
Conclusion: Embracing AI for a Smarter Supply Chain
For innovators crossing the chasm, AI-driven supply chain automation is the key to unlocking efficiency, agility, and resilience. While challenges such as data quality, system integration, and organizational resistance are real, they are not insurmountable. By taking a strategic approach — focusing on incremental improvements, leveraging AI tools, and fostering a culture of innovation — businesses can overcome these obstacles and fully realize the potential of AI in their supply chains.
The future of supply chain automation is AI-powered, and those who embrace this transformation will be better positioned to navigate the complexities of the modern global marketplace. For businesses ready to innovate, the time to act is now.