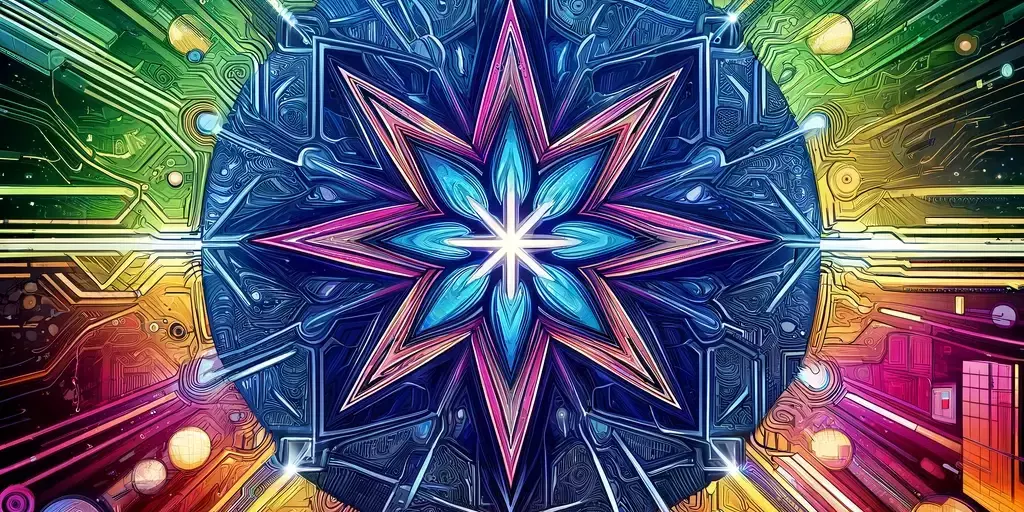
Generative AI models are at the forefront of technological innovation, offering capabilities that go beyond traditional AI. These models are designed to create new content, simulate real-world scenarios, and enhance various business operations. In this blog, we explore the main focuses of Generative AI models, highlighting their unique features and transformative potential.
Understanding Generative AI Models
Generative AI refers to AI systems that can generate new data or content from existing data. Unlike traditional AI, which primarily focuses on analyzing and predicting based on input data, Generative AI models create something new. These models use deep learning techniques, such as Generative Adversarial Networks (GANs), Variational Autoencoders (VAEs), and transformers, to learn patterns and generate content that is often indistinguishable from human-made content.
Main Focuses of Generative AI Models
1. Content Creation
Generative AI excels in creating various forms of content, including text, images, music, and videos. This capability is revolutionizing industries like marketing, entertainment, and media by automating content production and enhancing creative processes.
Examples:
- Text Generation: AI models like GPT‑4 can write articles, stories, and even code.
- Image Generation: Tools like DALL·E create unique images from text descriptions.
- Music Composition: AI can generate original music tracks tailored to specific styles or moods.
Impact:
- Speeds up content production
- Reduces costs associated with manual creation
- Enables mass customization
2. Data Augmentation
Generative AI is instrumental in augmenting datasets, especially when real-world data is scarce or difficult to obtain. This is particularly useful in training other AI models, as it helps create robust and diverse datasets.
Examples:
- Synthetic Data Generation: AI models generate synthetic data for training machine learning models, enhancing their performance and reliability.
- Simulation: AI simulates various scenarios for testing and development, such as virtual environments for autonomous vehicles.
Impact:
- Improves model training accuracy
- Enhances data diversity
- Reduces dependency on real-world data
3. Personalization
Generative AI can create highly personalized experiences for users by analyzing their preferences and generating tailored content. This focus is crucial in industries like e‑commerce, healthcare, and entertainment, where personalization drives user engagement and satisfaction.
Examples:
- Product Recommendations: AI generates personalized shopping recommendations based on user behavior.
- Healthcare: AI creates personalized treatment plans by analyzing patient data and predicting responses to treatments.
Impact:
- Enhances user engagement
- Increases customer satisfaction
- Boosts conversion rates
4. Design and Prototyping
Generative AI accelerates the design and prototyping process by generating multiple design iterations quickly. This focus is particularly valuable in industries such as automotive, aerospace, and architecture, where design efficiency is crucial.
Examples:
- Automotive Design: AI generates various car design prototypes, optimizing for aerodynamics and aesthetics.
- Architectural Design: AI creates multiple building designs based on specified parameters, streamlining the planning process.
Impact:
- Speeds up the design process
- Reduces development costs
- Enhances innovation and creativity
5. Enhancing Decision-Making
Generative AI aids in decision-making by generating potential scenarios and outcomes based on existing data. This focus is vital for strategic planning and risk management across various industries.
Examples:
- Financial Modeling: AI generates financial forecasts and risk assessments, aiding in investment decisions.
- Supply Chain Management: AI simulates supply chain disruptions and generates strategies to mitigate risks.
Impact:
- Improves decision-making accuracy
- Enhances strategic planning
- Reduces risks and uncertainties
Challenges and Future Directions
Despite its transformative potential, Generative AI faces several challenges, including ethical considerations, data privacy, and the need for substantial computational resources. Addressing these challenges will be crucial for the continued development and adoption of Generative AI technologies.
Future Directions:
- Ethical AI: Developing guidelines and frameworks to ensure the ethical use of Generative AI.
- Improved Models: Enhancing the accuracy and efficiency of Generative AI models.
- Wider Applications: Expanding the use of Generative AI across more industries and applications.
Conclusion
Generative AI models are revolutionizing various industries by focusing on content creation, data augmentation, personalization, design, and decision-making. As these models continue to evolve, their applications and impact will only expand, driving innovation and efficiency. By understanding and leveraging the main focuses of Generative AI, businesses can unlock new opportunities and stay ahead in the competitive landscape.